Operation Performance Management Primer: From Performance Measures to Performance Management
Chapter 3. Operations Performance Management: Major Functions
Overview
The major functions of an OPMM program are discussed in this chapter. OPMM’s major functions are as follows.
- Performance Measurement. OPMM measures are clearly defined and are easy to understand and visualize by technical and nontechnical audiences. They provide decision support for the operation of specific TSMO activities (e.g., the need for signal timing plan changes), describe multimodal performance, and are directly applicable for making decisions. At least some measures focus on performance from the customer’s/traveler’s point of view; measures are continuously reviewed and modified. High-quality data are available and data sources are standardized and integrated across all department/agencies; long-term budget commitments for the purchase of private data are established.
- Performance Monitoring. A single data system houses all operations data, which are seamlessly integrated; the system is routinely updated and modified using sound Information Technology (IT) principles; agency personnel receive ongoing training on the use of the system; hardware architectures and technology routinely upgraded to improve performance; systems and data integration/interoperability maintained on a continuing basis across multiple agencies in the region, and real-time multimodal situational awareness actively used to support adaptive multi-agency operational plans.
- Reporting. OPMM performance measures are reported internally for utilization and externally for accountability and program justification. Reporting systems automatically flag areas where performance does not meet targets or expectations; operations performance measures are used in general agency planning and programming activities.
- Project/Strategy Evaluation. A routine and ongoing evaluation of TSMO projects is established and uses data from the single data system with little or no need for additional data collection. Before/after analysis of completed projects is done with controls to ensure that background factors are not affecting treatment outcomes. When projects produce positive results, they are highlighted in communication and public relations instruments for decisionmakers and the public. When projects do not produce the desired outcomes, after-action analysis is conducted to determine why.
- Operate. OPMM is fully integrated into all related agency functions and used to create planning and programming documents on equal footing with all other functional areas. OPMM strategic, tactical, and program plans are integrated with jurisdictions’ multisectoral plans and programs (e.g., Performance-Based Practical Design, long-range transportation programs, and the Congestion Management Process) based on formal continuing planning processes. OPMM goals and objectives are included in general planning documents. The OPMM program leads to TSMO strategies being considered simultaneously with capacity and demand management strategies at all stages of project development (planning, preliminary engineering, design). Operations data is used to diagnose congestion problems and assess impacts of all types of strategies.
- Input for Investment Decisionmaking. In addition to routine reporting of performance, decisions on TSMO investments should be informed by performance measures. Performance measures are used to describe the size and nature of mobility problems in such a way that TSMO strategies can be tailored to address them.
Performance Measurement
System monitoring and performance measurement are important components of OPMM.13 These components are interrelated components as performance measures indicate how well the transportation system is performing while monitoring refers to tracking performance over time. In addition to providing a means of monitoring progress towards operations objectives, performance measures also allow an agency to:
- Identify needs and system performance deficiencies.
- Assess potential impacts of TSMO strategies.
- Evaluate effects of implemented projects.
- Communicate progress to stakeholders.
Monitoring helps transportation agencies to reach better decisions about how to operate the system and the investments needed to support operations. With the information gathered from performance measurement and monitoring tasks, an agency can gain a better understanding of system performance needs and deficiencies, measure progress towards operational objectives, and ultimately refine project and program implementation and operation. That same data generated from the OPMM approach can even be fed back into the planning process for a fully integrated approach to transportation decisionmaking. However, without a mechanism to measure and monitor performance, an agency will not realize the full value of developing operational objectives.
To get started, agencies should follow these steps to develop the performance monitoring function of OPMM:
- Where should monitoring take place? Should we monitor facilities, entire trips, or both? How should facilities and trips be defined (what is their extent)? Do we only monitor facilities and trips we know are already congested or do we include the entire system under our purview?
- What should be monitored? What aspects of congestion and safety should we monitor: do we monitor not only congestion level but also its determinants like demand and disruptions? What performance measures should we use for each of these categories?
- When should monitoring occur? What time periods should be monitored: weekday peak periods, off-peak periods, and/or weekends?
- How will monitoring be achieved? What data are available for supporting the desired monitoring activity? Will new data collection have to be performed? What analytic tool will be used to perform the monitoring? Can the analytics be performed in-house, by a commercial tool, by a custom-built system, or by a service contract?
- Who will be responsible for managing the monitoring portion of OPMM activities? What staff will be involved?
Operations Performance Measures
Overview
TSMO covers a wide range of activities including the management of incidents, weather, work zones, traffic control devices, traveler information, and traffic flow (e.g., Active Traffic Management). Operations performance measures should be constructed for each of these program areas. The Federal Highway Administration (FHWA) and transportation agencies have constructed a broad list of performance measures that can be applied for OPMM.14
Conceptually, performance measures for OPMM are organized around the factors that influence congestion and safety as shown in figure 3. For example, consider traffic incident factors. The “first order” cause of incident-related congestion is the amount of time that lanes and shoulders are blocked, which in turn are influenced by several factors. Continuing down the chain, one of the factors, incident duration, is a function of many subfactors, including the effectiveness with which incidents are managed. This framework is considered when constructing OPMM performance measures. These measures have the following general features.
Mobility Measures
For the purpose of OPMM, mobility measures are those that relate to the travel time experience of transportation system users. This experience can be demonstrated for individual highway facilities and trips made by any mode. Users’ travel time experience can also be summarized by region and State.
Mobility measures have a long history in the profession, but only within the past decade has data been available to permit measuring systemwide performance.15 Data availability played a key role in the recent creation of Federal mobility performance measures. Based on the MAP‑21 legislation, FHWA promulgated rules regarding mobility, and developed the “third performance management rulemaking (PM3)” that must be reported by State DOTs and MPOs.16 Three measures were developed:17
- National Highway Performance Program Reliability: The percent of the National Highway System that is deemed to be reliable.18
- Freight Reliability: Truck travel time reliability index for the Interstate system.19
- Annual Hours of Peak Hour Excessive Delay per Capita: For selected urbanized areas, the total amount of person delay per person. (National Performance Measures for Congestion Mitigation and Air Quality Improvement Program—Traffic Congestion).20
To support agencies’ reporting requirements, FHWA also made available a data set, the National Performance Management Research Data Set that is to be used not only for Federal reporting requirements but for other OPMM activities as well.21
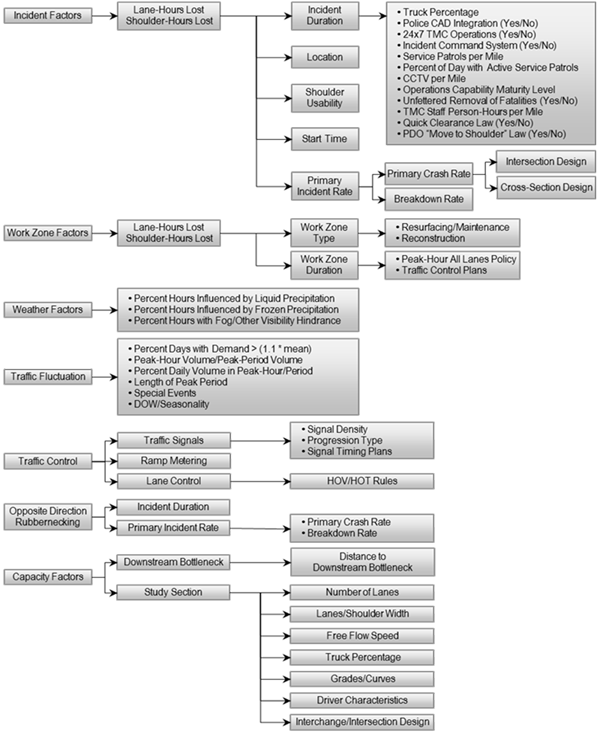
Figure 3. Flow chart. Influencing factors for congestion.
(Source: Federal Highway Administration.)
A flow chart showing how the primary sources of congestion and reliability (incidents, weather, work zones, demand, traffic control, and capacity) are in turn influenced by specific factors. For example, incident effects are determined by the duration of lane and shoulder blockages. These are influenced by how frequently incidents occur, how long they last, and where they occur on the network. All of these factors are affected by incident management strategies. Similar influencing “chains” exist for the other sources of congestion.
The PM3 are constructed to gauge systemwide mobility performance. Many other measures have been developed that are better suited for measuring the performance of individual facilities, trips, and bottlenecks. For example, FHWA has fostered the use of reliability measures by practitioners even before the PM3 were developed.22 Travel time reliability is how mobility performance of a facility, trip, or system varies from day-to-day. It is influenced by the variability in system conditions: incidents, inclement weather, work zones, and demand that change over time. Reliability is, therefore, extremely important for OPMM. Other examples of operations performance measures follow:
The FHWA has recommended performance measures for monitoring freight bottlenecks:23, 24
- Total Delay (vehicle-hours and person-hours)—Actual vehicle-hours (or person-hours) experienced in the highway section minus the vehicle-hours (or person-hours) that would be experienced at the reference speed. Delay can be compared across the system when normalized by segment length to get total delay per mile.
- Hours of delay per truck-vehicle-hours of delay normalized by number of trucks.
- Mean Travel Time Index—The mean travel time over the highway section divided by the travel time that would occur at the reference speed.
- Planning Time Index—The 95th percentile Travel Time Index computed as the 95th percentile travel time divided by the travel time that would occur at the reference speed.
- 80th Percentile Travel Time Index—The 80th percentile Travel Time Index computed as the 80th percentile travel time divided by the travel time that would occur at the reference speed.
- Hours of Congestion per Year—Number of hours where vehicle speeds are below predetermined thresholds.
- Average and 95th Percentile Queue Length—Developed from a distribution of queue lengths, the highway distance where the speeds of contiguous segments upstream of an identified bottleneck location are less than a threshold.
- Value of wasted time and fuel due to congestion for each segment—Calculated as congestion delay multiplied by the value of time and the value of excess fuel consumption.
External Factors Affecting Mobility
Traffic demand (volumes) is a highly significant form of data for OPMM. Demand not only drives the congestion produced by physical bottlenecks, but determines the severity of disruptions such as traffic incidents and work zones; under light traffic conditions, an incident or work zone may have no appreciable impact on traffic flow. Previous work showed how small changes in vehicle-miles traveled (VMT) (a common measure of demand) can have large impacts of performance measures.25 Table 1 shows how performance degraded slightly between 2006 and 2007 with only a marginal increase in VMT. However, performance improved significantly between 2007 and 2008 when VMT dropped due to the onset of the economic recession. In this case, a 3.1 percent drop in VMT led to a 12 percent decrease in average travel times and a 10 percent decrease in the 95th percentile travel time.
Table 1. Changes in performance on selected Atlanta, Georgia freeways, 2006 to 2008.
Metrics |
Year |
2006 |
2007 |
2008 |
Travel Time Index |
1.720 |
1.800 |
1.585 |
Average Travel Time |
10.033 |
10.492 |
9.220 |
95thPercentile Travel Time |
14.266 |
15 row3.151 |
13.597 |
80thPercentile Travel Time |
11.874 |
12.400 |
10.989 |
Skew Statistic |
1.186 |
1.196 |
1.308 |
Daily Vehicle Miles Traveled |
1,789,122 |
1,790,030 |
1,734,742 |
(Source: Cambridge Systematics et al. (2013). Analytical Procedures for Determining the Impacts of Reliability Mitigation Strategies, Strategic Highway Research Program 2 Report S2‑L03‑RR‑1, Transportation Research Board, Washington, D.C.)
Demand data that is continuously collected is the richest type of data not only for real-time TSMO applications, but for OPMM; continuous demand data allows for the creation of demand profiles that can be used in modeling. Further, demand should be used in creating certain OPMM performance measures (e.g., delay) and in weighting facility- and trip-based statistics for aggregation to areawide statistics. Demand data is collected continuously from freeway detectors as well as some from signalized highway detectors as discussed earlier in this chapter. FHWA has produced material useful for understanding and assessing external influences on OPMM performance measures.26
Additionally, traffic volume data are most often included in the statewide roadway inventory maintained by each State DOT. The most common traffic volume attribute in a statewide roadway inventory is annual average daily traffic, which is a single average count value for each calendar year. More detailed traffic count data are available from permanent continuous count stations, but these detailed counts are not available for the entire roadway network, only a limited number of locations.
At the program level, understanding trends in demand—as well as in traffic disruptions—helps to explain trends in outcome measures. As part of transparency and accountability reporting, simply reporting trends in outcomes measures (e.g., delay and reliability) is insufficient. Displaying how outcome measures change over time tells us whether conditions are better or worse, but they do not tell us why they are better or worse. Understanding the factors that led to outcome trends is significant for a number of reasons:
- Knowledge of the relative contribution of the factors will lead to better investment selection at the project level (“targeted investments”) and better definition of programs at the statewide level (e.g., more emphasis on one of the factors).
- Understanding how external factors affect performance is extremely useful for setting performance targets. For example, forecasts of areawide economic factors are widely available, and understanding how these factors affect performance will aid agencies in determining the most appropriate targets.
- Understanding the effects of external factors on performance outcomes allows agencies to identify the causes of dips or spikes in performance that may be surprising, to “adjust” trends (i.e., to produce a more normalized time series) based on what-if assumptions, and to facilitate cross-sectional comparison of performance outcomes among locales and corridors over a given period of time.
- Communicating mobility performance to management and the public is enhanced with a deeper understanding of why trends occurred. Being able to explain how external factors, especially ones that are largely outside of the control of transportation agencies, influence trends demonstrate transparency and can also be used to help justify program investments. It also provides professionals with hard information rather than relying on anecdotes and guesses when communicating mobility in a journalistic sense.
Two Strategic Highway Research Program 2 (SHRP 2) projects can help practitioners understand the influence that external factors have on mobility in general and reliability specifically. SHRP 2 Project L11, Evaluating Alternative Operations Strategies to Improve Travel Time Reliability, explored the influence of several factors:27
- Demographics, land use, and urbanization.
- Environment and climate change.
- Energy costs and availability.
- Technological innovation.
- Freight trends.
- Finance, road pricing, and privatization.
SHRP 2 project L04 examined how external factors influencing reliability can be included in forecasting models.28
Figure 4 demonstrates the concept of how external factors affect demand and the other contributing factors to congestion, the “first order” determinants of mobility: incidents, weather, work zones, capacity, and demand.
It is clear that demand has the greatest number of external influencing factors and the effect of demand on OPMM performance measures cannot be overstated. Demand can be changed in a number of ways: the total number of trips made, trip length, or temporal distribution of trips (e.g., peak versus off peak). Accounting for how external factors affect each of these aspects of demand is a critical component of any relationships that are developed.
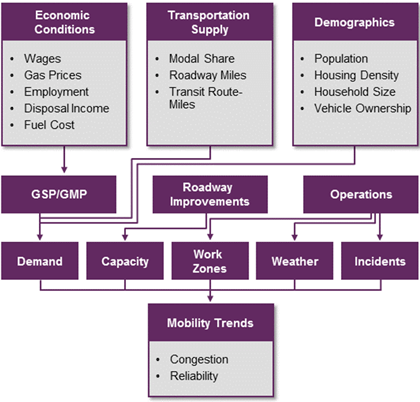
Figure 4. Flow chart. Relationship between external factors and operations performance measures and management.
(Source: Federal Highway Administration.)
A flow chart showing that economic, infrastructure (roadway supply), and demographic conditions affect the primary sources of congestion (incidents, weather, work zones, demand, and capacity). Economic and demographic conditions drive travel demand. Roadway improvements and operations affect roadway supply. All of these factors interact to produce mobility and reliability conditions that travelers experience.
Performance Monitoring
Collecting data on actual system performance allows a region to monitor performance trends and determine if indicators are moving in the right direction. Performance measures and trends can then be communicated internally and externally through periodic performance reports. Periodic performance reports are an effective tool for communicating how the OPMM approach is used to improve performance and the quality of life for the traveling public. A number of agencies already use performance reports to inform decisionmakers about system performance and the effectiveness of OPMM strategies.
Reporting
Periodic performance reports support the OPMM approach in a number of ways including:
- Providing a realistic view of system performance improvements that can be attained through management and operational investments.
- Providing operations managers with up-to-date benchmarks on how, or if, operations programs are contributing to performance objectives.
- Supporting realistic policies that recognize system constraints to performance improvement and also the role of system management and operations to maintaining a desired level of performance.
Related to the discussion in the Planning for Operations section, periodic performance reporting is best supported through sustained communication between planning and operations staffs. Sustained communication encourages routine coordination between staffs and allows operational tools to be more effectively leveraged during the planning process. Thus, in addition to being a core component of OPMM, periodic performance reporting supports the fundamental concept of planning for operations on which OPMM relies.
The FHWA has compiled numerous examples on reporting congestion and safety performance.29 Beyond these, several other reporting mechanisms offer insight into how to conduct performance reporting:
- Figure 5 is an example of statewide reporting of travel time reliability, showing the trends over time. Trend analysis is highly useful for gauging the top-level effects of OPMM and other congestion mitigation programs. Increasing unreliable travel is an indicator that TSMO strategies could be beneficial.
- Figure 6 shows how congestion on a facility grows and dissipates over time and space. This chart may be produced in real time or may use monthly or annual averages. This visual analysis communicates the nature and extent of congestion on a facility.
- Figure 7a is a facility-level diagram showing the reliability of the facility with several causal factors of reliability shown. One can see the high variability in travel times on this facility through the travel time distribution, which is the basis for developing reliability performance measures. This type of diagnostic “drills down” into the root causes of congestion and can be used to target TSMO strategies.
- Figure 7b is another example of presenting facility-level performance in times of several performance measures.
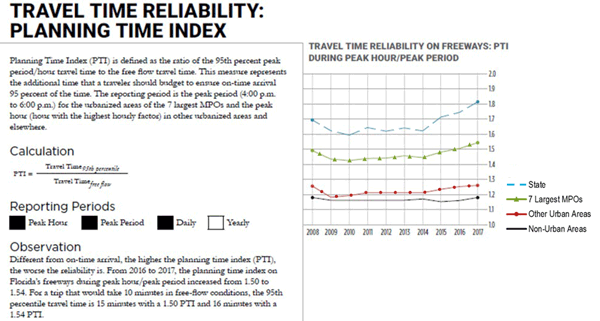
Figure 5. Chart. Travel time reliability trends on Florida freeways.
(Source: Florida Department of Transportation, 2020.)
An example of statewide reporting of travel time reliability, showing the trends over time. Trend analysis is highly useful for gauging the top-level effects of OPMM and other congestion mitigation programs. Increasing unreliable travel is an indicator that TSMO strategies could be beneficial. Specifically, this figure shows the Planning Time Index increasing over time in Florida, especially in major urban areas.
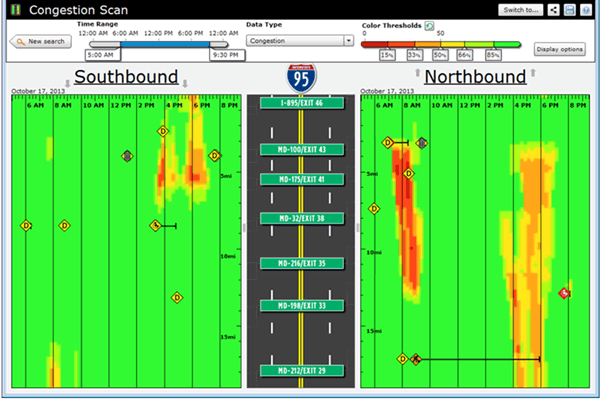
Figure 6. Screenshot. Depiction of congestion in time and space.
(Source: University of Maryland Center for Advanced Transportation Technology Lab, 2018.)
A dashboard showing how congestion on a facility grows and dissipates over time and space. This chart may be produced in real time or may use monthly or annual averages. This visual analysis communicates the nature and extent of congestion on a facility.
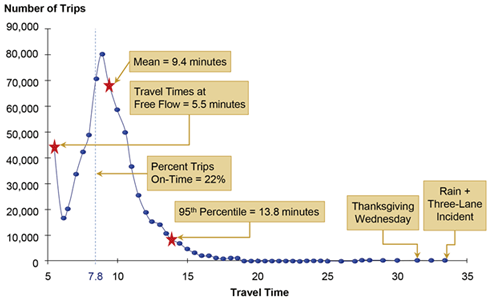
Figure 7a. Chart. Reliability characteristics: I‑75 Northbound I‑85 Roswell Road, Atlanta.
(Source: Federal Highway Administration, 2021.)
Line chart showing a frequency distribution of travel times. It starts with travel times at free flow at a frequency of around 45,000 at 5.5 minutes, the mean around 70,000 at 9.4 minutes, the 95th percentile around 10,000 at 13.8 minutes, Thanksgiving Wednesday at zero at 31.5 minutes, and rain and three lane incident also at zero at around 33.5 minutes. The percent trips on-time = 22%.
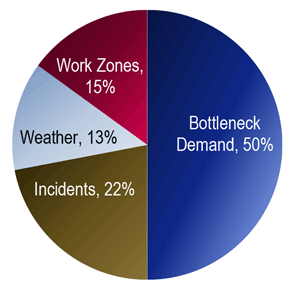
Figure 7b. Chart. Sources of Congestion: I‑75 Northbound I‑85 Roswell Road, Atlanta.
(Source: Federal Highway Administration, 2021.)
Table 2a. Time slice—PM peak period (4:30 p.m. to 6:30 p.m.).
Disruption |
Type |
Indicator |
Incident |
13.9 Lane-Hours Lost (LHL) |
Work Zone |
8.8 LHL |
Percent Days Weather Influenced |
11% |
Percent Days with Demand >110% off Normal |
18% |
Table 2b. Incident analysis.
Category |
Number |
Duration (Minutes) |
Mean |
95th Percentile |
All |
94 |
32.1 |
75.5 |
Lane-Blocking |
90 |
32.4 |
75.5 |
Large Truck |
2 |
20.3 |
38.6 |
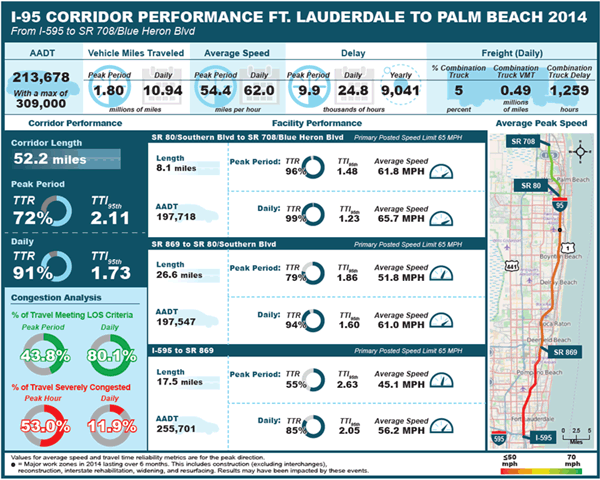
Figure 8. Screenshot. Presentation of travel time measures in on a facility.
(Source: Florida Department of Transportation.)
Project/Strategy Evaluation
Overview
Evaluation is an important step in a management-based approach to operations that is closely tied to monitoring/measurement activities. Evaluation refers to the practice of assessing the effectiveness of an operational strategy or practice towards achieving a desired performance objective. It is closely tied to monitoring/measurement activities because without the measurement of performance and monitoring performance indicators over time, evaluation is not possible.
Assessing the effectiveness of strategies and investment through evaluation studies is necessary if an agency is to realize the full benefits of OPMM. Having a better understanding of the effectiveness of strategies and investments allows transportation planners and operators to work together to devise more effective investment strategies to meet performance objectives. Strategies that are already working can be fine-tuned to perform even better while those that are not working can be fixed or abandoned.
Evaluation is closely tied to monitoring/measurement through three interrelated elements as part of an iterative process:
- Assessing the effectiveness of strategies.
- Tracking system performance.
- Refining operations objectives.
As discussed at the beginning of this chapter, assessing the effectiveness of strategies provides agencies the opportunity to further refine those strategies or to devise new ones. It also provides the necessary input to the Reporting element of OPMM so that agencies may be able to communicate the value of various operational strategies to decisionmakers and the public. Importantly, to be able to assess the effectiveness of various strategies, agencies will need to have data on before-and-after conditions. Otherwise, there is no baseline by which to measure whether performance has improved or worsened.
A key component of OPMM is the ability to conduct evaluations of completed projects and to use the results to make better-informed investment decisions in the future. Historically, evaluation of congestion mitigation projects, including TSMO, were limited in number and scope because of data scarcity. Conversely, transportation agencies routinely invest great effort in forecasting the impact of transportation improvements, but practitioners have seldom had the opportunity to evaluate the outcomes of these investments, except in isolated cases where special studies are conducted. The large amount of data that is now available allows evaluations to become routine with little or no additional data collection needed.
Although a rich history of evaluations has been accumulated, the vast majority of studies lack a consistent method; common performance measures; and, most problematic, controls for dealing with factors that can influence the observed performance other than the project treatment.30 A thorough treatment of an evaluation methodology is beyond the scope of this primer. Regardless of what a comprehensive methodology entails, at its core, it will be examining travel time and demand data. The remainder of this section focuses on the use of these data types.
Relevant Data
A comprehensive and statistically valid evaluation methodology will have to draw on a variety of data types to establish controls. Observational studies usually make use of experimental controls to account for the effect of variation in the measurement of interest and for the effect of exogenous factors. In classic observational before and after studies, controls are sites that have not received the improvement (treatment), but have underlying characteristics similar to the test sites before the improvement. Figure 9 shows an example of tracking congestion via the Travel Time Index over time for a control and test (treatment) site.
Because the primary impact of interest is congestion, another method of establishing experimental controls can also be used. This approach is based on examining the underlying causal factors to congestion in the before and after periods. Travel time-based impacts are the primary factor of interest, but many factors can influence travel time besides an improvement project, including incidents, demand (e.g., day-to-day and seasonal variations and special events), weather, work zones, traffic controls, and general operations policies. Ideally, the influence of these factors is stable (or nearly so) in the before and after periods of project implementation, which allows for observed changes in travel times to be untainted. In theory, all of these data types can be obtained from archived operations data, although in practice, some operations may choose not to archive certain types of data.
Analytical Procedures
Figure 10 outlines an approach for evaluation TSMO (or any congestion mitigation) projects. The concept is to quantify the factors that can influence travel times in the before and after periods, decide if they are roughly equivalent, and if not, make adjustments to the travel time measures based on applying models. Using models to adjust the controls has not been examined deeply yet, but it is similar to the Empirical Bayes method used in highway safety analysis, where observed data is adjusted for external influences using a model.31
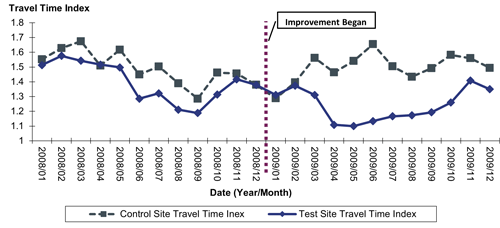
Figure 9. Line graph. Example time plot of travel time index on test and control sites.
(Source: Federal Highway Administration.)
An example of tracking congestion via the Travel Time Index over time for a control and test (treatment) site. In this example, the control and treatment sites show the same pattern in the before period. In the after period, the treatment site showed an improvement in congestion for the first six months but then showed slowly worsening conditions in the second six months.
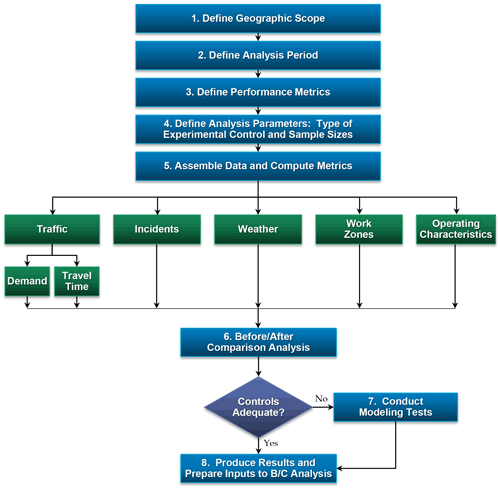
Figure 10. Flow chart. Before/after evaluation methodology using controls for exogenous factors.
(Source: Federal Highway Administration.)
Operate
Up to this point, the OPMM discussion in this Primer has been on monitoring and understanding past trends in mobility, the effectiveness of TSMO strategies to address them, and using this information so that state and local transportation agencies can invest more effectively. Most TSMO strategies are dynamic in that they adapt to current conditions, so OPMM has a real-time as well as a historical context. Decision support systems (DSS) are a key tool in implementing dynamic control of TSMO strategies. DSS use a variety of data sources in an automated, computer-based environment to support business and operational decisionmaking. Applications of DSS include Integrated Corridor Management where a key aim is to balance demand with available capacity, including the consideration of multiple modes.
As with historical OPMM activities, DSS rely on performance measurement of the transportation system, but in a real-time situation. Emerging nontraditional sources of data enable new insights into system performance, and can lead to identifying previously undetected trends and relationships.