Traffic Analysis Toolbox Volume XII:
Work Zone Traffic Analysis – Applications and Decision Framework
Chapter 4. Key Considerations for Modeling Approach
4.1 Introduction
After a suitable analysis tool is selected, the next step is to use the tool to determine the impacts of a work zone. This chapter describes the key considerations when applying the modeling tools for work zone traffic analysis.
Before proceeding to discuss each category of the modeling tools, it is important to have an understanding of the following key items, as they play a significant role in work zone analysis when using any of the modeling tools.
- Study Network;
- Work Zone Capacity;
- Capacity versus Discharge Rate;
- Variability of Capacity and Time-of-Day Models;
- Analysis Period;
- Change in Traffic Pattern;
- Common Data Collection;
- Model Development Application Process;
- Error Checking; and
- Level of Effort.
Study Network
In preparation for the data collection required for work zone analysis, it is necessary to determine the limits of the network to be studied. The study network should consider both the work zone boundaries and the surrounding area where the traffic is affected by the work zone. Specifically, the following should be considered:
- The network boundary should be able to accommodate queues under the worst scenario, including the work zone conditions;
- Include all detour routes, if possible;
- For freeways and highways, include on- and off-ramps, and other routes to which traffic is diverted; and
- For arterials, consider the following:
- Include, at a minimum, the adjacent intersections both upstream and downstream of the work zone;
- Include all intersections outside of the work zone that are impacted by queues from intersections within the work zone;
- Consider the limits of the coordinated signal system; and
- Consider including any side streets that are expected to be impacted by work zone queues.
For instance, to obtain an initial estimation of the queue length caused by the work zone at signalized intersections, the following equation may be used: (Work Zone Analysis Guide. Maryland State Highway Administration (SHA), Maryland, September 2008. Accessed January 11, 2012.)

Where:
L = The approach queue length (in number of vehicles);
V = The through traffic volume for the approach;
G = The duration of the green interval for the through movement;
C = The cycle length;
N = The number of open through lanes; and
s = The saturation flow rate (in vehicles per hour per lane (vphpl)). Local data at work zones shall be used. In the absence of better local data, the analyst may use 1,400 vphpl (1,200 to 1,600 vphpl) as the starting point and make modifications accordingly to reflect local work zone conditions.
If the work zone will directly impact more than one intersection, the queue length at the critical intersection, and at the first intersection that motorists will encounter while traveling through the work zone should be evaluated. The critical intersection is defined as the intersection where one or more of its approaches impacted by the work zone have the highest existing volume per through lane. Figure 11 illustrates how to determine where the queue length should be evaluated. (Work Zone Analysis Guide. Maryland State Highway Administration (SHA), Maryland, September 2008. Accessed January 11, 2012.)
Figure 11. Locations of Queue Length Evaluation
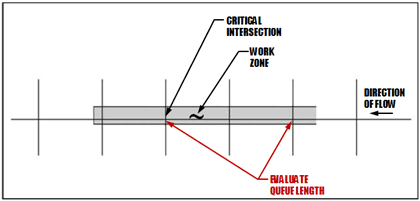
(Source: Maryland State Highway Administration, 2008.)
Work Zone Capacity
The Highway Capacity Manual 2010 (HCM 2010) defines capacity as “the maximum sustainable hourly flow rate at which persons or vehicles reasonably can be expected to traverse a point or a uniform segment of a lane or roadway during a given time period under prevailing roadway, environmental, traffic, and control conditions.” (Highway Capacity Manual 2010 (HCM 2010). Transportation Research Board, National Research Council, Washington, D.C., 2011.) Roadway capacity in a work zone is reduced due to a variety of factors, including:
- Work zone speed;
- Work intensity (work zone type);
- Percentages of trucks;
- Number of lanes;
- Number of lane closures;
- Lane width;
- Lateral clearance;
- Work zone layout (lane merging, lane shifting, and crossover);
- Length of closure;
- Presence of ramps;
- Work zone location (urban or rural);
- Work zone duration (long-term or short-term);
- Work time (daytime or nighttime);
- Work day (weekday or weekend);
- Type of control devices and their placement;
- Weather conditions (sunny, rainy, or snowy);
- Pavement conditions (dry, wet, or icy);
- Pavement grade; and
- Driver composition (commuters or non-commuters, such as tourists).
Tables 27 and 28 list work zone lane capacity defaults for use (in passenger cars per hour per lane or pcphpl) based on previous studies, with Table 27 for short-term work zones and Table 28 for long-term ones. (Highway Capacity Manual 2010 (HCM 2010). Transportation Research Board, National Research Council, Washington, D.C., 2011. Weng, J., and Q. Meng. A Decision Tree-Based Model for Work Zone Capacity Estimation. The 90th TRB Annual Meeting, National Research Council, Washington, D.C., January 2011. WSDOT Work Zone Safety and Mobility (in Design Manual). Washington State Department of Transportation, December 2009.) It is strongly recommended, though, that local data at work zones are collected and used instead. In the absence of better local data, the analyst may use these defaults as the starting point and make modifications accordingly to reflect local work zone conditions. Also, some state DOTs and other agencies may have their own guidelines on work zone capacity and related defaults.
Table 27. Work Zone Lane Capacity Defaults that Can Be Used in the Absence of Better Data: Short-Term Work Zones
Short-Term Work Zone Capacity (pcphpl)
(Weng, J., and Q. Meng. A Decision Tree-Based Model for Work Zone Capacity Estimation. The 90th TRB Annual Meeting, National Research Council, Washington, D.C., January 2011. WSDOT Work Zone Safety and Mobility (in Design Manual). Washington State Department of Transportation, December 2009.) |
Freeway/Highway2 |
Arterial |
Urban |
Rural |
1,200-1,600 |
600
(These are average capacity values. The actual values would be dependent on several factors, which include the existing number of lanes, number of lanes closed, traffic speed, truck percentage, interchanges/intersections, type of work, type of traffic control, and seasonal factors (among others).) |
400
(These are average capacity values. The actual values would be dependent on several factors, which include the existing number of lanes, number of lanes closed, traffic speed, truck percentage, interchanges/intersections, type of work, type of traffic control, and seasonal factors (among others).) |
(Source: Transportation Research Board, 2011. Weng and Meng, 2011. WSDOT Work Zone Safety and Mobility, 2009.)
Table 28. Work Zone Lane Capacity Defaults that Can Be Used in the Absence of Better Data: Long-Term Work Zones
Note: Capacities through long-term construction zones are highly variable and depend upon many site-specific characteristics. It is, therefore, better to base this adjustment on local data and experience. If such data do not exist, and cannot be reasonably acquired, the range above may be used to provide a very approximate estimate of construction zone capacity.
Default Type |
Long-Term Work Zone Capacity (pcphpl)
(Highway Capacity Manual 2010 (HCM 2010). Transportation Research Board, National Research Council, Washington, D.C., 2011.)
Normal Lanes to Reduced Lanes (Freeways) |
2-1 |
3-2 |
3-1 |
4-3 |
4-2 |
4-1 |
Range |
1,200-1,800 |
1,300-1,600 |
1,200-1,800 |
1,300-1,600 |
1,300-1,600 |
1,200-1,600 |
Single Value |
1,400 |
1,450 |
1,450 |
1,500 |
1,450 |
1,350 |
(Source: Transportation Research Board, 2011. Weng and Meng, 2011. WSDOT Work Zone Safety and Mobility, 2009.)
Capacity versus Discharge Rate
Queue discharge flow rate represents traffic flow that has just passed through a bottleneck and is accelerating back to the free-flow speed (FFS). Queue discharge flow is relatively stable as long as there is not another nearby bottleneck downstream. The Highway Capacity Manual 2010 suggests that the queue discharge flow rate is lower than the maximum flows observed before breakdown (capacity). Two methods have been widely used in previous studies to define work zone capacity:
- Work Zone Capacity – Method 1 – The observable maximum throughput under relatively free-flow conditions before the formation of a traffic queue; and
- Work Zone Capacity – Method 2 – The discharge flow rate under the queued conditions.
Method 1 is particularly relevant to traffic mitigation efforts to avoid queue formation and Method 2 is particularly relevant to manage queues, estimate the length of queues, and understand the amount of delay when upstream flow rates are consistently above the capacity of the work zone.
Since traffic conditions vary between pre-queuing and queuing conditions at work zone locations, a more precise work zone analysis may consider using both of the above work zone capacity values: one for pre-queuing conditions, and the other for queuing conditions. However, if resources or schedules do not allow for the development of separate models using different capacity values for pre-queuing and queuing conditions, select one of the above methods using engineering judgment and previous experience. The analyst may concentrate on other more important factors that have bigger impacts on model calibration and alternatives analysis.
Variability of Capacity and Time-of-Day Models
Roadway capacity within a work zone may vary due to the following factors:
- Driver Characteristics – This may vary from hour to hour, from day to day, and from one region to another. This variation is mainly due to the ability of the driver population to adapt to the changing conditions. For instance, Al-Kaisy et al. suggested that commuter drivers are expected to be more familiar with the route and the work zone configuration. (Al-Kaisy, A., M. Zhou, and F. Hall. New Insights into Freeway Capacity at Work Zones: Empirical Case Study. In Transportation Research Record 1710, Transportation Research Board, National Research Council, Washington, D.C., 2000, pages 154-160.) Therefore, they can proceed through the work zone with shorter headways and higher flows. Similarly, Al-Kaisy and Hall examined the effect of driver population at freeway reconstruction zones. (Al-Kaisy, A., and F. Hall. Examination of Effect of Driver Population at Freeway Reconstruction Zones. In Transportation Research Record 1776,Transportation Research Board, National Research Council, Washington, D.C., 2001, pages 35-42.) Based on a factor of 1.0 for commuter traffic, a driver population factor of 0.93 was estimated for the afternoon peak period and a driver population factor of 0.84 was estimated for weekends. The driver population factor was responsible for more of a capacity reduction on weekends compared with the capacity on weekdays. It should be noted that the reduction factor varies and it depends on local conditions.
- Nonrecurrent Events – Nonrecurrent events such as inclement weather and traffic accidents further reduce capacity within a work zone. For instance, in the HCM 2010 (Exhibit 10-15), inclement weather may reduce the capacity of freeways by 1 percent to 22 percent, depending on the intensity of the weather condition.
- Work zone Duration – The duration of the work zone has an impact on driver behavior; after a period of time, drivers adjust to the changed conditions and capacity and speeds may go up. According to Kim, Lovell, and Paracha, the average capacity at long-term work zones was noticeably greater than that at short-term ones, as drivers became more familiar with the work zones. Based on this study, long-term work zones may have capacities as much as 150 pcphpl higher than short-term ones. (Kim, T., D.J. Lovell, and J. Paracha. A New Methodology to Estimate Capacity for Freeway Work Zones. The 80th TRB Annual Meeting, National Research Council, Washington, D.C., Paper No. 01-0566, January 2001.) It should be noted that the capacity difference between long-term and short-term work zones varies and it depends on local conditions.
- Random Effect – Even when all prevailing conditions are held constant, capacity was found to be a random variable, because of stochastic variations in vehicle mix, lane distribution, and driver behavior. (Horowitz, A. Microsimulation of Freeway Work Zones to Assess Flow and Capacity. University of Wisconsin-Milwaukee, Milwaukee, Wisconsin, 2009.)
As discussed above, work zone capacity is influenced by many factors. Therefore, sensitivity analysis may be deemed necessary when resources allow.
Analysis Period
Work zone capacity is influenced by many factors; it may be specific to different times of the day, different demographic groups (age/experience), and different populations reflecting regional or local tendencies. Therefore, if different time-of-day analysis is considered critical and resources are available, it is recommended to create different time-of-day models, such as a.m. peak, off-peak, p.m. peak, weekend peak, and weekend off-peak, to reflect the variable capacity, as well as the variable demand in analysis tools. Also, for longer duration work zones, it is recommended that interim analysis be performed to better reflect varying capacities, if resources and data are available.
For longer duration work zones, it is recommended that interim analysis be performed to better reflect varying capacities, if resources and data are available.
In addition, different analysis tools may accommodate various time periods; some can analyze longer time periods, while others cannot. For instance, some sketch-planning analysis tools can analyze longer time periods, such as multiple days, months, or even years, which is typically beyond the capability of other analysis tools such as macro-, meso-, and microscopic simulation models.
The analysis period should be long enough to include no queue at the beginning, queue buildup, and queue dissipation at the end of the analysis period.
Changes in Traffic Pattern
Drivers obtain roadway information through various means to select their preferred route(s). These means include dynamic message signs (DMS), highway advisory radio (HAR), public information and outreach, incident management systems or other traveler information systems, etc. Some tools, such as DynusT, have the ability to directly model roadway information system, such as DMS. In DynusT, DMS can be set to provide multiple types of messages, such as speed advisory, mandatory detour, and congestion warning. DynusT permits users to specify a percentage of vehicles that respond to the DMS and make detour decisions.
According to Horowitz et al., many drivers are responsive to warnings that they might encounter excessive delays along their current route. (Horowitz, A.J., I. Weisser, and T. Notbohm. Diversion from a Rural Work Zone Owing to a Traffic-Responsive Variable Message Signage System. Accessed January 11, 2012.) However, a much larger percentage of drivers would not divert, even though it is to their advantage to do so. This study suggested that a 10 percent alternative route selection rate during peak periods is achievable when accurate, up-to-the-minute information about delay through a work zone is provided and there is an attractive set of alternative routes. This study was conducted on a rural freeway. For drivers using lower classification or urban highways with attractive alternative routes, the diversion rate could be higher.
The FHWA conducted a study that examined the use of ITS for work zone traffic management with a purpose to highlight “before and after” or “with and without” analyses that quantify the mobility and safety benefits. (Luttrell, T., M. Robinson, J. Rephlo, R. Haas, J. Srour, R. Benekohal, J. Oh, and T. Scriba. Comparative Analysis Report: The Benefits of Using Intelligent Transportation Systems in Work Zones. Publication FHWA-HOP-09-002, Federal Highway Administration, U.S. Department of Transportation, Washington, D.C., October 2008.) According to this study, significant traffic diversion was observed in response to appropriate messages displayed during congested conditions. In Texas, an average of 10 percent diversion (range of 1 to 28 percent) was observed, while in the District of Columbia (D.C.) an average of 52 percent (range of 3 to 90 percent) lower mainline volume (combination of diversion, demand reduction, and congestion) was observed. The study noted that in Texas, during major incidents or high construction impact periods combined with high demand, the system diverted an average of 10 percent of mainline traffic to alternate routes and traffic diversion was as high as 28 percent.
When congestion occurs on the mainline due to a work zone, motorists may choose alternate routes to bypass the congested segment. Subsequently, travel time on the alternate routes will increase, and the route choice between the mainline and an alternate route may vary over time. Dynamic traffic assignment (DTA)-based tools can take this into account. In the case of long-term work zones, generally traffic reaches equilibrium after a few weeks, especially in urban areas with commuter traffic. Travelers typically choose the available route having the least travel time between their origin and destination (O-D). Due to congestion effects, the travel time of a route between an origin and destination also depends on the choices made by other travelers, who are themselves also trying to choose the least travel time route between their own origin and destination. When every traveler succeeds in finding such a route, every used route has the minimum time or cost between its origin and destination. This condition is known as equilibrium. The collection of local data is encouraged in order to create a more robust analysis network that captures the effects of changes in traffic pattern.
Besides finding alternate routes, some motorists may change their trip departure times or even cancel their trips, especially in the case of long-term work zones with heavy congestion.
Common Data Collection
Once the size of the study network has been determined, it is necessary to collect data that will be needed to model work zone operations. The data collection effort may be considerably reduced by obtaining information using aerial imagery, or by downloading the traffic signal plans from agency web sites, and/or by obtaining the roadway design plans (if available). If needed, these data should be confirmed with a field visit.
It should be noted that the required data accuracy may vary during different project stages (e.g., project planning, preliminary engineering, and final design). It is not appropriate to use planning-level data to make engineering design decisions.
Included in Table 29 are the common data that should be collected for all work zone traffic analysis, no matter which method or tool is selected. Additional data for specific modeling tools will be discussed in each tool category. Depending on the complexity of the study network and of the analysis needed, different types of data may be required and may broadly be classified into four categories: general, geometric, traffic, and construction.
Table 29. Work Zone Data Collection – Common Data
Category |
Data |
General |
- Location of work zone
- Weather conditions
- Police presence
- Flagger presence
- Crash/incident information
- Traffic impact mitigation strategies
|
Geometry |
- Lane width
- Number of lanes in each direction
- Number of open lanes
- Presence of ramps
- Length of lane closure
- Position of closed lane
- Length of work activity
- Turn bay lengths
- Mid-block intersections
|
Traffic |
- Volume of traffic
- Heavy vehicle percentage
- Volume of pedestrians
- Speed limits
- Speed of vehicles in work zone
- Locations of traffic control devices
- Signal detection type and location
- Signal phasing and timing
- Queue lengths
- Presence of congestion (bottlenecks) – location and time of day
- Length and duration of congestion
- Driver behavior
- Travel time
- Delay
- Saturation flow rate
- Growth factor
- Seasonal variation factor
|
Construction |
- Type of construction activity
- Number of workers present
- Number and size of construction equipment
- Proximity of work activity to the travel lanes in use
- Traffic control devices used
|
Origin-Destination Data
Origin-destination (O-D) data is often needed for work zone traffic analysis. This data can be used to serve as a basis for trip assignment in the existing conditions models and trip redistribution between alternate routes in the analysis models. O-D data may be estimated based on a select link analysis using a regional travel demand model, field observations/studies, and data from the private sector such as INRIX® and TomTom®. The preferred method of obtaining origin-destination data is a field study, such as a post card survey or license plate study, as the travel demand model may not have the fidelity to generate an accurate estimate for detailed traffic operations analysis. For less complex projects, origin-destination data may not need to be collected. Instead, assumptions may be made based on engineering judgment as to reasonable traffic distribution and traffic trends. Additionally, the appropriate agency may be consulted for assistance with traffic distribution and diversion assumptions. More information on performing O-D studies can be found in the Institute of Transportation Engineers’ (ITE) Manual of Transportation Engineering Studies.
Origin-Destination Matrix Estimation (ODME)
The use of an Origin-Destination matrix is fundamental to the application of a travel demand model. However, the development of that O-D matrix can be both difficult and costly due to the need for extensive traveler survey data. An O-D matrix may be available or easily made available from the local MPO or other transportation planning agency. In addition, by using traffic counts and other available data, it may be possible to estimate a “reasonable” O-D matrix. This process is called ODME.
Many estimation techniques exist for developing a reliable O-D matrix, including Least Squares (constrained or not), statistical methods such as Likelihood methods and Kalman filtering, Bayesian estimator or combined estimators and Moving Horizon Estimation (MHE) methods.
Model Development and Application Process
In order to evaluate the impacts of a proposed work zone, it is necessary to build models for both existing and work zone conditions. The existing conditions or preconstruction model can be built using data collected for existing conditions of the study network. This may include the existing lane configurations, existing traffic volumes, and existing signal timings and phasing. The model is then calibrated to represent field observed conditions through the adjustment of parameters in the analysis tool. This calibrated existing conditions model, often called the “no-build” model, provides the baseline for the performance of the roadway facility. The existing conditions model can then be modified to represent work zone conditions.
The basic principles of work zone traffic analysis tools vary from one type to the next. The overall procedure of developing and applying models to work zone analysis consists of the following major tasks. A flowchart, complementing the overall process, is presented in Figure 12. It should be noted that the existing conditions (“no-build”) model development and calibration may be skipped if there already is a calibrated model available. The available existing conditions model could be generated by a project leading to the work zone analysis or from other independent studies.
- Identification of Project Scope and Data Collection – The project scope needs to be identified to define the study objectives, breadth (geographic and temporal boundaries), approach, tools, resources, and time schedule for the study. Once the project scope is determined, data should be collected for model development and calibration, such as roadway geometry, traffic demands, capacities, traffic controls, travel times, and queues.
- Existing Conditions Model Development – Once data are collected, the next step is to develop an existing conditions model with the correct geometry, traffic demands, capacities, and traffic controls. Coding errors can distort the subsequent model calibration process and cause the analyst to adopt incorrect values for the calibration parameters. Therefore, error checking, as described in the next section, should be performed to identify and correct those model coding errors. If a calibrated existing conditions model already is available from other resources, this step and the following existing conditions model calibration step can be skipped.
- Existing Conditions Model Calibration – Each analysis tool has a set of user-adjustable parameters that enable the analyst to calibrate the tool to better match specific local conditions. This calibration process involves the selection of a few parameters for calibration and the repeated operation of the model to identify the best values for those parameters. The calibration process can be time-consuming. It should be well documented so that later reviewers of the model can understand the rationale for the various parameter changes made during calibration.
- Work Zone Base Conditions Model Development – The next step is to develop a work zone base conditions model by modifying the calibrated existing conditions model to represent work zone conditions. These modifications may include geometry, traffic demands, capacities, and traffic controls. Roadway geometry and traffic controls can usually be collected from construction drawings or field surveys. Traffic demands for the work zone base scenario are usually generated from travel demand models, traffic counts, or O-D surveys. The preferred method of obtaining traffic demand data is a field study, as the travel demand model may not have the fidelity to generate an accurate estimate for detailed traffic operations analysis.
- Work Zone Base Conditions Model Calibration – Work zone model calibration may include the calibration of both work zone capacity and performance measures. The work zone capacity calibration process is tool-dependent; some tools allow direct changes to capacity, while others do not have a “capacity” input and require adjusting a set of parameters in the tool that affect capacity. The performance measures collected either from the field or based on prior experience and engineering judgment, such as queue lengths, travel times, delays, and speeds, also must be calibrated in the model. Typically, work zone analysis is performed to gain insight into the potential impact of the proposed work zones. Therefore, collecting field data for the work zone to be implemented will likely not be possible. Under this case, the analyst should analyze similar work zone types in the project area to better understand local driving behaviors and implement those in the model.
- Alternatives Analysis – Significant efforts have been made on developing and calibrating the model. Now it is time to put the model to work by analyzing various project alternatives. The alternatives analysis may involve the forecasting of the future demand and the testing of various project alternatives against the base case. The analyst needs to run the model for each alternative, review the output, compare against any mobility requirements, extract relevant statistics, and perform various analyses of the results. Some agencies may have their own mobility criteria. For instance, MD SHA uses LOS, control delay, and travel time as mobility requirements and the thresholds for arterials. (Work Zone Analysis Guide. Maryland State Highway Administration (SHA), Maryland, September 2008. Accessed January 11, 2012.)
- Final Report/Technical Documentation – The analytical results are summarized in a final report and the analytical approaches are documented in a technical document. This task also may include presentation of study results to technical supervisors, elected officials, and the general public.
Figure 12. Typical Model Development and ApplicationProcess for Work Zone Analysis
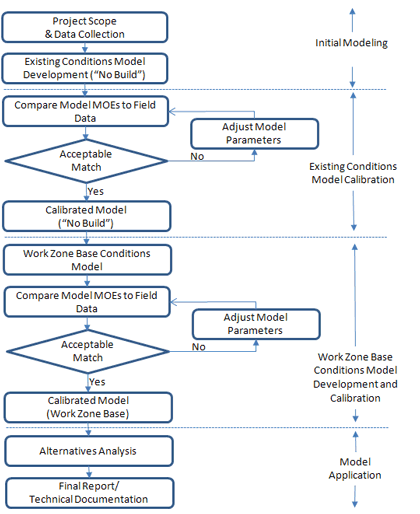
Error Checking
The error-checking step is necessary to identify and correct model coding errors so that they do not interfere with the model calibration task. Coding errors can distort the model calibration process and cause the analyst to adopt incorrect values for the calibration parameters. Error checking involves various tests of the coded network and the demand data to identify input coding errors. The goal of the error-checking step is to eliminate all major errors in network coding and demand before model calibration.
Error checking involves various reviews of the coded network and coded demands. Error checking proceeds in the following basic stages:
- Review Software Errors – The analyst should review the software and user group web sites to ensure that he or she is aware of the latest known “bugs” and user workarounds for the software. The analyst should ensure that he or she is using the latest “patch” of the software.
- Review Coded Network – The analyst should review the coded network, including:
- Check network connectivity.
- Check geometry, including lengths, number of lanes, free-flow speed, facility type, etc. Overlaying the coded network over aerial photographs of the study area is a quick way to verify the accuracy of the coded network geometry. In addition, color codes may be used to help identify network links with different attributes.
- Check intersection controls, including control type and control data, if there are intersections in the network.
- Check lane closures or restrictions.
- Review Demand Data – The analyst should review the demand data, including:
- Check vehicle inputs or O-Ds;
- Check vehicle mix proportions; and
- Verify output volumes against traffic counts.
- Other Reviews – There are some other review items that are tool-specific. For instance, microsimulation models require animation review to spot less obvious input errors. Animation output enables the analyst to see the vehicle behavior that is being modeled and assess the reasonableness of the microsimulation model itself. Running the simulation model and reviewing the animation, even with artificial demands, can be useful to identify input coding errors.
Level of Effort (LOE)
The level of effort on conducting work zone traffic analysis varies with analysis tool types. In general, sketch-planning tools require the lowest level of effort, while microscopic simulation tools demand the highest level of effort.
The level of effort determination typically provides labor-hour estimates for project tasks. These estimates assist project managers and program leaders in developing budget and schedule estimates, as well as the scope of the project. Project tasks typically incorporated into the level of effort labor-hour estimates of a traffic analysis project include:
- Data collection;
- Base model and calibration;
- Alternatives analysis;
- Documentation/presentations; and
- Project management.
For additional information regarding the development of a level of effort especially for a traffic analysis project using microsimulation, please refer to the FHWA sponsored Guidance on the Level of Effort Required to Conduct Traffic Analysis Using Microsimulation. (Work Zone Analysis Guide. Maryland State Highway Administration (SHA), Maryland, September 2008. Accessed January 11, 2012.)
In the subsequent sections, some of the commonly used software packages are mentioned in each tool category, and parameters are suggested to adjust for work zone analysis. Please note that these are associated with the current software versions, and future software updates may result in different parameters. Please refer to the latest documentation of the software for the most up-to-date interpretation of those parameters.
4.2 Using Sketch-Planning and Analytical/Deterministic Analysis
Sketch-planning and analytical/deterministic analysis tools (HCM-based) are typically specialized models designed for a specific application, such as work zone analysis. These types of tools are in contrast to other general-purpose tools, such as travel demand models and microscopic simulation models, which are targeted towards a much wider range of application, not just work zone analysis. Sketch-planning and analytical/deterministic analysis tools include a wide range of software, including spreadsheet models developed by individuals or state DOTs for specific projects or conditions, to more generalized delay estimation tools such as QuickZone and QUEWZ-98. They vary in complexity from analyzing only individual roadway segments to network-based analyses. Often, they are based on simple queuing techniques or volume-to-capacity relationships from the HCM.
The key strength of sketch-planning and analytical/deterministic analysis tools is their relative ease of use and ability to facilitate a rapid analysis. Typically, a sketch-planning or analytical/deterministic tool requires fewer resources and less staff training since they are simpler in terms of data requirements, calibration, and interpretation of the results. An analysis using a sketch-planning or analytical/deterministic tool is usually quite rapid. This is important in cases where a decision needs to be made quickly or the agency desires a less resource-intensive analysis. In addition, some sketch-planning analysis tools can analyze longer time periods, such as multiple days, months, or even years, which is typically beyond the capability of other analysis tools such as macro-, meso-, and microscopic simulation models.
Some of the challenges of sketch-planning and analytical/deterministic analysis tools include limited network complexity and presentation capabilities, and the high level of the analysis techniques. For example, QUEWZ-98 only allows simple “pipeline” analysis without the ability to model cross-streets and detour routes. The results from sketch-planning and analytical/deterministic analysis tool are relatively high-level (e.g., average queue and maximum queue) and the results are constrained by the quality of the input data and analysis methodology. Sketch-planning and analytical/deterministic analysis tools are primarily used in the planning stages of a project, and are not considered to be a substitute for the detailed engineering analysis often needed later in the project implementation process.
Some examples of sketch-planning and analytical/deterministic analysis tools are listed below. For a complete list, please refer to Traffic Analysis Toolbox Volume I: Traffic Analysis Tools Primer. (Alexiadis, V., K. Jeannotte, and A. Chandra. Traffic Analysis Toolbox Volume I: Traffic Analysis Tools Primer. Publication FHWA-HRT-04-038, Federal Highway Administration, U.S. Department of Transportation, Washington, D.C., June 2004.)
The computational engine, FREEVAL (FREeway EVALuation) 2010 as shown in Figure 13, is a computerized, work sheet-based environment designed to implementthe operational analysis computations for undersaturated and oversaturated directional freeway facilities. (Rouphail, N., B. Schroeder, and B. Eads. HCM2010 Chapter 10 Freeway Facilities User’s Guide to FREEVAL2010. February 2011.) It incorporates entire freeway segment procedures outlined in Chapters 11, 12, and 13 in HCM2010 for basic freeway segments, weaving segments, and merge and diverge segments, respectively. FREEVAL-2010 is executed in Microsoft Excel, with most computations embedded in Visual Basic modules. It allows the user to analyze a freeway facility of up to 70 analysis segments and for up to 24, 15-minute time intervals (six hours). The engine can generally handle any facility that falls within these temporal and spatial constraints. It provides clean outputs, such as summary work sheets with segment performance (e.g., speed, density, volume, volume-to-capacity ratio (v/c), demand-to-capacity ratio (d/c), and LOS, and 3-D contour plots. FREEVAL-2010 can handle both undersaturated (d/c ≤ 1.0) and oversaturated traffic conditions (d/c > 1.0). When the facility is considered to be “oversaturated,” an additional output, i.e., demand-based LOS, is generated.
FREEVAL-2010 is not a commercial software product; instead, it is made available freely to all HCM users in HCM2010s on-line Volume 4. FREEVAL-2010 allows the user to make changes to geometry, segment capacity, demand patterns, and free-flow speeds to analyze the operational effects of work zones.
Figure 13. FREEVAL-2010
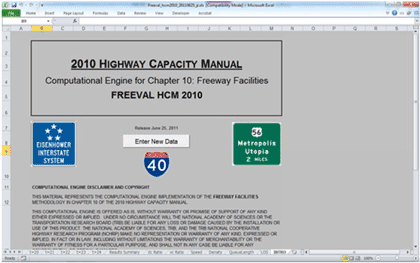
(Source: Rouphail, Schroeder, and Eads, 2011.)
Data Collection
The precise input data required by a sketch-planning or analytical/deterministic analysis tool will vary by software and the specific modeling application as defined by the study objectives and scope. Most sketch-planning and analytical/deterministic analysis tools require the following types of input data, besides the general data identified at the beginning of this chapter.
Existing Models
Check if the agency maintains a database of sketch-planning and analytical/deterministic analysis model files. A field visit may need to be performed to verify the accuracy of the model input data.
Traffic Volumes
Most sketch-planning and analytical/deterministic analysis tools require traffic volumes as input data. Traffic volumes can be either collected in the field, be extracted from existing detectors (if available), or exported from other analysis tools. Also, procedures exist in many demand models to generate O-D tables for the study area, which can be easily converted to traffic volumes.
Calibration Data
Calibration data consist of measures of traffic counts and system performance such as speeds and queues.
Other Data
Some sketch-planning and analytical/deterministic analysis tools such as QuickZone require detailed work zone project data, such as project start date, project duration, and construction phases.
Capacity in Modeling Tools
At the beginning of this chapter, details on work zone lane capacity were presented. It is strongly recommended that local data at work zones be collected and used, as driver characteristics vary from region to region and capacity may vary from location to location. In the absence of better local data, the analyst may use the capacity defaults as the starting point and make modifications accordingly to reflect local work zone conditions.
Capacity can be directly specified in most sketch-planning and analytical/deterministic analysis tools. Typically, the user can adjust the capacity for any type of link in the network, if needed. In addition, the free-flow speed (if appropriate) can be modified as well for capacity calibration. Table 30 lists major parameters that the analyst may adjust for work zone analysis in several commonly used sketch-planning and analytical/deterministic analysis tools.
Table 30. Parameters to Adjust for Work Zone Analysis in Common Sketch-Planning and Analytical/Deterministic Analysis Tools
Sketch-Planning and Analytical/Deterministic Analysis Tools |
Primary Parameters |
Secondary Parameters |
FREEVAL |
- Capacity Adjustment Factors
- Free-Flow Speed
- Number of Lanes
|
- Traffic Composition (Percent Trucks)
|
HCS |
- Capacity
- Free-Flow Speed
- Number of Lanes
|
|
IDAS |
- Lane Capacity
- Free-Flow Speed
- Number of Lanes
|
|
QUEWZ-98 |
- Work Zone Capacity
- Number of Lanes Closed
- Length of Lane Closure
- Schedule of Work Activity
|
- Percentage of Trucks
- Speed-Volume Relationship
- Definition of Excessive Queuing
|
QuickZone |
- Capacity
- Number of Lanes
- Free-Flow Speed
- Percent of Local Traffic Traveling on Detours (Percent)
|
- Truck Percentage
- Yearly Capacity Decrease (Percent)
- Yearly Demand Increase (Percent, for Large Projects that Extend over Several Years)
- Mode Shift Change (Percent)
- Cancel Trip (Percent)
|
Key Considerations Regarding Use for Analysis
The key strengths of sketch-planning and analytical/deterministic analysis tools are their relative ease of use and ability to facilitate a rapid analysis. This is important in cases where a decision needs to be made quickly or the agency desires a less resource-intensive analysis. Some sketch-planning tools can analyze a longer time span in one model, such as multiple days, months, or even years of work zones, which is typically beyond the capability of other analysis tools such as macro-, meso-, and microscopic simulation models. Discussed below is the model development and application process when using analytical/deterministic analysis tools.
Model Development and Application Process
The typical model development and application process, which was discussed at the beginning of this chapter, applies to sketch-planning and analytical/deterministic analysis tools as well. Compared to other types of tools, the model calibration of sketch-planning and analytical/deterministic analysis tools is more high-level and less time-consuming.
Sketch-Planning Model Development and Application – Check List
This subsection provides a high-level synthesis combining the concepts presented above on model development and application. Please note that not all the items listed below are required for all work zone analysis.
- Determine Study Objectives – The goals should directly correlate to the work zone safety, mobility, constructability concerns, and needs of the agency. The performance objectives then determine the selection of work zone alternatives or strategies that are designed to meet the established goals.
- Identify Needed Coordination Tasks – Determine what coordination must occur between other projects, agencies, facilities, and events. This input may require additional modeling to quantify impacts, such as when work is to occur on an arterial that contains mass transit.
- Determine Study Network – Determine the limits of the study network. The network boundary should be able to accommodate queue lengths under the worst scenario, including the work zone conditions. The network also should include potential detour routes, both signed and unsigned.
- Determine Analysis Time Period – Determine the analysis time period. If needed, create different time-of-day models, such as a.m. peak, off-peak, and p.m. peaks. For longer duration work zones, it is recommended that interim analysis be performed to better reflect varying capacities, if resources and data are available. Each analysis period should be long enough to include no queue at the beginning, queue buildup, and queue dissipation at the end of the analysis period.
- Confirm Analysis Method – Verify that the analysis tool(s) selected have sufficient capabilities to satisfy the analysis needs with regards to work zone characteristics, transportation management plan, data, resources, and performance measures.
- Select Measures of Effectiveness and Thresholds – The MOEs or thresholds selected will be customized to the agency’s goals and project characteristics. The MOEs and thresholds set a minimum performance standard that the alternatives must meet or exceed.
- Collect and Analyze Data – Collect data that is needed to model work zone operations. Depending on the complexity of the study network and of the analysis needed, different types of data may be required. Data may broadly be classified into four categories: general, geometric, traffic, and construction.
- Identify Work Zone Alternatives – Identify potential work zone alternatives for evaluation.
- Conduct Fatal Flaw Analysis – Perform a fatal flaw analysis on each selected alternative to determine the viability of the alternative for further analysis.
- Determine Work Zone Capacity – Roadway capacity in a work zone is reduced due to a variety of factors. It is strongly recommended that local data at work zones be collected and used. Check with the appropriate stakeholder agencies as some of them may have their own guidelines on work zone capacity and related defaults. In the absence of better local data, the analyst may use the proposed defaults as the starting point and make modifications accordingly to reflect local work zone conditions.
- Develop Existing Conditions Model – Develop an existing conditions model with the correct geometry, traffic demands, capacities, and traffic controls. Error checking should be performed to identify and correct the model coding errors.
- Calibrate Existing Conditions Model – Calibrate the model by adjusting a set of user-adjustable parameters in the analysis tool to better match specific local conditions.
- Develop Work Zone Base Conditions Model – Develop a work zone base conditions model by modifying the calibrated existing conditions model to represent work zone conditions.
- Calibrate Work Zone Base Conditions Model – Work zone model calibration includes the calibration of both work zone capacity and performance measures.
- Conduct Alternative Analysis – The alternatives analysis may involve the forecasting of the future demand and the testing of various project alternatives against the base case. The analyst needs to run the model for each alternative, review the output, compare against any performance requirements, extract relevant statistics, and perform various analyses of the results.
- Recommend Best Alternative – Utilizing a decision framework, the best overall alternative is selected.
- Document Findings – The analytical results are summarized in a final report and the analytical approaches are documented in a technical document.
4.3 Using Travel Demand Model Analysis
Travel demand models are analytical models that forecast future travel demand based on current conditions, and future projections of household and employment characteristics. Traditionally, they are large regional planning models used by metropolitan planning organizations (MPO), and were originally developed to determine the benefits and impacts of major highway improvements in metropolitan areas. A distinguishing feature of travel demand models is their geographic coverage, which generally includes an entire metropolitan area: a city, its suburbs, and the adjacent counties.
For work zone analysis, a key strength in using travel demand models is their ability to predict areawide traffic redistribution. For example, if an agency is considering closing an important urban freeway-to-freeway interchange for several months while it is rebuilt, a travel demand model could help evaluate the overall changes in total daily traffic volumes on various roadways throughout the region. In general, travel demand models can handle larger networks more efficiently than mesoscopic and microscopic simulation models. Another important consideration is that most major metropolitan areas already have a travel demand model available that could be used either as the sole analysis tool or as a foundation for developing a transportation network or forecasting travel demand for another model type (e.g., mesoscopic and microscopic simulation models).
However, travel demand models have limited capabilities to accurately estimate changes in operational characteristics (such as speed, delay, and queuing) resulting from the implementation of operational strategies and changes. This includes the effects associated with roadwork construction. Because these models are prepared at a broad regional scale, they lack detail that may be critical to the analysis objective. For example, they may model only one or two time periods (such as the a.m. peak hour or the daily average), which may not be sufficient for analyzing time-specific work zone traffic management strategies.
Some examples of travel demand models are listed below. For a complete list, please refer to Traffic Analysis Toolbox Volume I: Traffic Analysis Tools Primer. (Alexiadis, V., K. Jeannotte, and A. Chandra. Traffic Analysis Toolbox Volume I: Traffic Analysis Tools Primer. Publication FHWA-HRT-04-038, Federal Highway Administration, U.S. Department of Transportation, Washington, D.C., June 2004.)
Data Collection
Besides the general data identified at the beginning of this chapter, travel demand models require the following types of input data.
Existing Models
Check if the MPO or other local transportation planning agency maintains a regional travel demand model. A field visit may need to be performed to verify the accuracy of the model input data. Additionally, since the regional travel demand model maintained by an agency typically covers a significant area, it may be necessary to have a subarea model created for use in work zone analysis (see Subarea Modeling in Key Considerations Regarding Use for Analysis).
Calibration Data (Traffic Counts and Speed/Travel Time)
For work zone analysis, calibration data typically consist of measures of traffic counts and speed/travel time.
Model Refinement Data (Traffic Analysis Zone Splitting)
Often the physical changes to the roadway associated with a work zone are localized in nature and, thus, only impact the physical characteristics of the corridor where the work zone is present. For larger projects, work zone mitigation strategies may result in physical changes to detour routes in addition to changes to the subject corridor.
Utilizing a travel demand model to capture the impact of a work zone requires the analysis of the localized corridor(s) where the work zone is occurring or has a significant impact, as opposed to regional travel patterns. As such, it may be necessary to increase the fidelity of the model by improving the network details surrounding the corridor(s) being studied. In addition, it may be necessary to improve details for parallel corridors if there are significant anticipated operational impacts or if potential mitigation strategies make significant changes to the physical or operational characteristics of these detour corridors.
A travel demand model typically represents travel in a region by generating trips from an origin to a destination, determining the mode split for these trips and then assigning these trips to the transportation network. The origin and destinations for these modeled trips are represented by Traffic Analysis Zones (TAZ). The size and complexity of these TAZ can vary by location in the model. Often, TAZ near the center (often the downtown area of a model) of the model are smaller in size and can represent a single city block or several city blocks combined. This TAZ will connect to the transportation network with several centroid connectors to allow modeled trips to be transferred from the TAZ to the represented transportation network. Further out toward the edges of the modeled area, TAZ often become larger and can represent several square miles of development with more limited number of centroid connectors. The size of TAZ represented near the corridor being studied should be examined to determine if they need to be split into smaller TAZ or if additional centroid connectors need to be added to the network to accurately represent travel near the corridor.
Capacity in Modeling Tools
At the beginning of this chapter, details on work zone lane capacity were presented. It is strongly recommended that local data at work zones are collected and used, as driver characteristics vary from region to region and capacity may vary from location to location. In the absence of better local data, the analyst may use the capacity defaults as the starting point and make modifications accordingly to reflect local work zone conditions.
Capacity can be directly specified in travel demand models. Typically, the user can adjust the lane capacity for any link type in the network to reflect any capacity reduction due to the work zone.
The major parameters that the analyst may adjust for work zone analysis using travel demand models include:
- Number of lanes;
- Free-flow speed; and
- Capacity (vehicles per hour per lane).
Key Considerations Regarding Use for Analysis
The main strengths of using travel demand models for work zone analysis are their ability to model large geographic areas and predict areawide traffic redistribution. Travel demand models, however, have limited capabilities to accurately estimate changes in detailed operational strategies (such as optimized signal operations). Therefore, it is important that the tool selection process detailed in Chapter 3 has been undertaken to ensure that travel demand models are the appropriate tool for the specific work zone analyzed. Discussed below are key considerations when using travel demand models, including:
- Subarea modeling;
- Link length change to match work zone area; and
- Model development and application process.
Subarea Modeling
Travel demand models generally cover large regional areas and are maintained by MPOs. Travel demand models do not have to be cut to smaller subareas for work zone modeling. However, sometimes it is necessary to do so for faster modeling and better focus on the work zone and its immediate vicinity. If a subarea model is created for a particular analysis, care should be taken to ensure that any potential detour or alternate routes be contained within the subarea so that impacts from the changes associated with a work zone can be accurately captured.
The subarea models are typically validated using observed hourly count data. An Origin Destination Matrix Estimation (ODME) process is typically applied to improve the match between model volumes and observed counts in the subarea.
Creating subarea models is necessary when travel demand models are used to generate Origin-Destination (O-D) tables for other tools such as microscopic simulation analysis which do not capture traffic diversion directly. The subarea selected corresponds to the area to be modeled in the microscopic simulation analysis. Often, the subarea model created from the travel demand model can be utilized as a basis for generating the roadway network to be simulated in the microsimulation. However, because the roadway networks generally utilized for travel demand modeling are developed for conventional static planning applications, they often lack several key features. Some of these missing or deficient data include:
- Time-Dependent Component for the O-D Demand – Commonly, the O-D demand is associated with a full 24-hour period or broken down into an a.m. peak, p.m. peak, and off-peak component. This fidelity may not be sufficient for detailed work zone analysis.
- Explicit Roadway Network Representation – Most travel demand applications only need the roadway network to represent the connections possible between links. Consequently, the actual roadway geometry may be approximated. For example, a highway interchange loop ramp may only be represented as a link connecting the freeway link to the arterial link and not represent the actual curving or loop geometry of the ramp.
- Signal Control Information – Signal controls are not typically explicitly modeled in a travel demand model. As such, signal timing and phasing data are not typically included.
The inclusion of this data often represents the differentiation between a travel demand model and a mesoscopic model.
The use of a travel demand model subarea as a starting place for the development of another simulation tool, such as microsimulation, calls for careful integration of several different data sources. When utilizing a travel demand model to support analysis from another simulation tool, the interface between the outputs of the travel demand model and the necessary inputs to the other simulation tool can vary widely. Depending on the models utilized, this link can vary from being nearly automated to very labor intensive. For example, the output of travel demand data from a VISUM travel demand model to a microsimulation of VISSIM is accomplished via a series of automated scripts that come as part of the PTV Simulation Suite. On the other extreme, the use of travel demand data for use in the microsimulation model CORSIM requires each input volume and turning assignment to be either manually input or a script must be written to accomplish this.
Link Length Change to Match Work Zone Area
Some links in travel demand models can be very long; it is not unusual to see links with a length of several miles. The work zone analyzed may be shorter than the corresponding link in the travel demand model. In this case, the link in the travel demand model has to be broken into shorter ones so that different link attributes (such as free-flow speed and capacity) can be assigned properly.
Model Development and Application Process
The typical model development and application process, which has been discussed at the beginning of this chapter, applies to travel demand models as well.
Travel demand models do not explicitly represent signal control at intersections. Less sophisticated travel demand models may utilize a simple volume-delay curve to represent the impedance of the signalized intersection on the approach links. The more sophisticated travel demand models utilize a “capacity calculator” to approximate the impact of signal control on the upstream links to the intersection. This capacity calculator applies HCM style intersection analysis to the turning movements calculated for each intersection. The results of this analysis are used to generate penalties for each movement, which are applied during the next iteration of the travel demand model.
- Travel demand models have a set of user-adjustable parameters that enable the analyst to calibrate the software to better match local conditions. This calibration process involves the selection of a few parameters for calibration and the repeated operation of the model to identify the best values for those parameters. The calibration process can be time-consuming. It should be well documented so that later reviewers of the model can understand the rationale for the various parameter changes made during calibration. For details on the calibration and validation process, please refer to the Model Validation and Reasonableness Checking Manual. (Barton-Aschman Associates, Inc., and Cambridge Systematics, Inc. Model Validation and Reasonableness Checking Manual. Travel Model Improvement Program, Federal Highway Administration, U.S. Department of Transportation, Washington, D.C., February 1997. Accessed January 11, 2012.)
- In most instances, it is beyond the scope of most work zone projects to undertake recalibration and validation of the regional travel demand model. There are typically strict calibration and validation guidelines associated with these models, which must be documented and verified by an independent agency. As such, this step is often skipped.
Travel Demand Model Development and Application – Checklist
This subsection provides a high-level synthesis combining the concepts presented above on model development and application for travel demand model use for work zone analysis. Please note that not all the items listed below are required for all work zone analysis.
- Determine Study Objectives – The goals should directly correlate to the work zone safety, mobility, constructability concerns, and needs of the agency. The performance objectives then determine the selection of work zone alternatives or strategies that are designed to meet the established goals.
- Identify Needed Coordination Tasks – Determine what coordination must occur between other projects, agencies, facilities, and events. This input may require additional modeling to quantify impacts, such as when work is to occur on an arterial that contains mass transit.
- Determine Study Network – Determine the limits of the study network. The network boundary should be able to accommodate queues under the worst scenario, including the work zone conditions. The network also should include potential detour routes, both signed and unsigned. For faster modeling and better focus on the work zone and its immediate vicinity areas, a subarea modeling may be necessary.
- Conduct Subarea Modeling – Determine whether a subarea model is necessary. Travel demand models do not have to be cut to smaller subareas for work zone modeling. However, sometimes, it is necessary to do so for faster modeling, better focus on the work zone and its immediate vicinity areas, and integration with other tools such as microsimulation analysis. Care should be taken to ensure that potential detour or alternate routes are contained within the subarea so that impacts from the changes associated with a work zone can be accurately captured.
- Split TAZ – The size of TAZ represented near the corridor being studied should be examined to determine if they need to be split into smaller TAZ or if additional centroid connectors need to be added to the network to accurately represent travel near the corridor.
- Determine Analysis Time Period – Determine the analysis time period. If needed, create different time-of-day models, such as a.m. peak, off-peak, and p.m. peak. For longer duration work zones, it is recommended that interim analysis be performed to better reflect varying capacities, if resources and data are available. Each analysis period should be long enough to include no queue at the beginning, queue buildup, and queue dissipation at the end of the analysis period.
- Confirm Analysis Method – Verify that the analysis tool(s) selected have sufficient capabilities to satisfy the analysis needs with regards to work zone characteristics, transportation management plan, data, resources, and performance measures.
- Select Measures of Effectiveness and Thresholds – The MOEs or thresholds selected will be customized to the agency’s goals and project characteristics. The MOEs and thresholds set a minimum performance standard that the alternatives must meet or exceed.
- Collect and Analyze Data – Collect data that are needed to model work zone operations. Depending on the complexity of the study network and of the analysis needed, different types of data may be required. Data may broadly be classified into four categories: general, geometric, traffic, and construction.
- Identify Work Zone Alternatives – Identify potential work zone alternatives for evaluation.
- Conduct Fatal Flaw Analysis – Perform a fatal flaw analysis on each selected alternative to determine the viability of the alternative for further analysis.
- Determine Work Zone Capacity – Roadway capacity in a work zone is reduced due to a variety of factors. It is strongly recommended that local data at work zones be collected and used. Check with the appropriate stakeholder agencies as some of them may have their own guidelines on work zone capacity and related defaults. In the absence of better local data, the analyst may use the proposed defaults as the starting point and make modifications accordingly to reflect local work zone conditions.
- Develop Existing Conditions Model – Develop an existing conditions model with the correct geometry, traffic demands, capacities, and traffic controls. Error checking should be performed to identify and correct the model coding errors.
- Calibrate Existing Conditions Model – Calibrate the model by adjusting a set of user-adjustable parameters in the analysis tool to better match specific local conditions.
- Develop Work Zone Base Conditions Model – Develop a work zone base conditions model by modifying the calibrated existing conditions model to represent work zone conditions.
- Change Link Length – The work zone analyzed may be shorter than the corresponding link in the travel demand model. In this case, the link in the travel demand model needs to be broken to shorter ones so that different link attributes (such as free-flow speed and capacity) can be assigned properly.
- Calibrate Work Zone Base Conditions Model – Work zone model calibration includes the calibration of both work zone capacity and performance measures.
- Conduct Alternative Analysis – The alternatives analysis may involve the forecasting of the future demand and the testing of various project alternatives against the base case. The analyst needs to run the model for each alternative, review the output, compare against any performance requirements, extract relevant statistics, and perform various analyses of the results.
- Recommend Best Alternative – Utilizing a decision framework, the best overall alternative is selected.
- Document Findings – The analytical results are summarized in a final report and the analytical approaches are documented in a technical document.
4.4 Using Traffic Signal Optimization Analysis
Traffic signal optimization tools are designed to develop optimal signal phasing and timing plans for isolated signalized intersections, arterial streets, or signalized networks. The more advanced traffic optimization tools are capable of modeling actuated and semi-actuated traffic signals, with or without signal coordination.
With respect to work zones, traffic signal optimization tools are useful when developing a signal plan for temporary traffic signal controls or analyzing signal plans when a detour route directs traffic to an existing signalized arterial roadway. The primary limitation of these tools is their single focus. Therefore, traffic signal optimization tools are typically used to provide supplementary analysis when analyzing the overall mobility impacts of a work zone. Signal optimization tools are sometimes combined with simulation tools to have a better understanding of traffic operations (both calibration of existing conditions models, as well as future alternatives analysis). Examples of this combination are Synchro/SimTraffic and TRANSYT-7F/VISSIM.
Some examples of traffic signal optimization tools are listed below. For a complete list, please refer to Traffic Analysis Toolbox Volume I: Traffic Analysis Tools Primer. (Alexiadis, V., K. Jeannotte, and A. Chandra. Traffic Analysis Toolbox Volume I: Traffic Analysis Tools Primer. Publication FHWA-HRT-04-038, Federal Highway Administration, U.S. Department of Transportation, Washington, D.C., June 2004.)
Data Collection
The precise input data required by a signal optimization model will vary by software and the specific modeling application as defined by the study objectives and scope. Besides the common data discussed at the beginning of this chapter, most signal optimization analytical work zone studies require the following types of input data.
Existing Models
Check if any of the local agencies maintain a database of traffic signal optimization model files. A field visit may need to be performed to verify the accuracy of the model input data.
Capacity in Modeling Tools
At the beginning of this chapter, details on work zone lane capacity were presented. It is strongly recommended that local data at work zones are collected and used, as driver characteristics vary from region to region and capacity may vary from location to location. In the absence of better local data, the analyst may use the capacity defaults as the starting point and make modifications accordingly to reflect local work zone conditions.
Once the work zone capacity is determined, the next step is to implement it in the signal optimization model. Among other things, the base saturation flow rate (SFR) is typically adjusted to represent the reduced capacity due to work zone operations. This can be accomplished either by reducing the lane widths/number of lanes through the work zone, or by changing the base saturation flow rate directly.
Table 31 lists some major parameters that the analyst may adjust for work zone analysis in several commonly used signal optimization software programs, including Synchro, Passer, and TRANSYT-7F.
Table 31. Parameters to Adjust for Work Zone Analysis in Common Signal Optimization Software
Signal Optimization Software |
Primary Parameters |
Secondary Parameters |
SYNCHRO |
- Number of lanes
- Ideal saturation flow rate
- Speed limit
- Lane inputs, including turn bays and U-turns
- Peak hour factor
|
|
PASSER |
- Number of lanes
- Ideal saturation flow rate
- Speed limit
- Lane inputs, including turn bays
- Peak hour factor
|
|
TRANSYT-7F |
- Number of lanes
- Base saturation flow rate
- Lane inputs, including turn bays
- Peak hour factor
|
- Heavy vehicle percentage
- Grade percentage
|
Key Considerations Regarding Use for Analysis
Signal optimization tools have a variety of applications for work zone analysis, especially in urban and suburban environments. Broadly speaking, these applications can be grouped in four categories:
- Preparing timing plans for temporary signals used to manage traffic within a work zone;
- Preparing timing plans for flagger or portable signals used to manage traffic within a work zone;
- Adjusting signal timing on corridors that are directly impacted by work zone; and
- Adjusting signal timing to improve progression on corridors that serve as alternate routes or detours around a work zone.
Model Development and Application Process
Signal optimization tools can be used to identify the need for temporary traffic signals and to revise the signal timing plans for existing signals where volumes are expected to increase or decrease as a result of the construction.
The typical model development and application process, which was discussed at the beginning of this chapter, applies to signal optimization tools as well. It should be noted that model calibration might not be required for some projects. If the analyst is unsure as to whether calibration will be required, consult with the contracting agency for a determination for the specific project.
For example, the Work Zone Analysis Guide by the MD SHA recommends the following guidelines be used to calibrate a Synchro model: (Work Zone Analysis Guide. Maryland State Highway Administration (SHA), Maryland, September 2008. Accessed January 11, 2012.)
- Synchro queue lengths should match field-measured queues on the critical movements within an 85 percent error (for field-measured queues shorter than 10 vehicles, a 2-vehicle error may be acceptable).
- Synchro travel times should match field-measured travel times within 85 percent. Note that the travel times should be compared for the overall length of the arterial, and not on a link-by-link basis.
- The SimTraffic simulations, a microsimulation tool coupled with Synchro, should match field-observed bottlenecks to the analysts’ satisfaction.
A work zone base conditions model is then developed by modifying the calibrated existing conditions model to represent work zone conditions. Adjustment to the base model to create the work zone base conditions model may include:
- Lane Configurations:
- Lane reductions on arterial segments can be modeled explicitly in most signal optimization tools. For instance, Synchro uses bend nodes and TRANSYT-7F uses dummy nodes to model lane reductions. Using Synchro as an example, bend nodes can be created on either end of the proposed work zone, overriding the number of lanes on one or both directions of the roadway. Take into consideration the required taper and buffer lengths approaching the work zone and adjust the model accordingly.
- When lane reductions occur through an intersection, take into consideration any impacts that this may have on the turning lanes from the side street(s).
- When lane and/or shoulder widths are reduced, the work zone capacity should be reduced accordingly.
- Traffic Volumes:
- When a detour route is being proposed, it will be necessary to redistribute the traffic volumes in the network based on O-D data and engineering judgment.
- If work zone demand management strategies are being employed, it may be necessary to reduce the traffic volumes by the estimated reduction in vehicle demand through the work zone.
- Saturation Flow Rate:
- Where there are no lane reductions, but where work would occur adjacent to the roadway, it may be necessary to adjust the saturation flow rate to represent the reduced capacity due to work zone operations. This can be accomplished either by reducing the lane widths through the work zone, or by changing the saturation flow rate directly.
For alternatives analysis, the analyst needs to run the model for each alternative, review the output, compare against any mobility requirements, extract relevant statistics, and perform various analyses of the results. Some agencies may have signal-related mobility criteria. For instance, MD SHA uses LOS, control delay, and travel time as mobility requirements, as listed in Table 32. (Work Zone Analysis Guide. Maryland State Highway Administration (SHA), Maryland, September 2008. Accessed January 11, 2012.)
Table 32. Mobility Thresholds for Arterials (MD SHA)
Signalized Intersections |
Existing LOS |
Mobility Threshold |
A, B, or C |
Maximum LOS D, control delay ≤ 45 seconds |
D |
Maximum increase in control delay of 30% |
E |
Maximum increase in control delay of 30%, or control delay ≤ 80 seconds |
F |
No increase in control delay |
Arterials |
TT |
Travel time cannot increase more than 15 minutes (maximum of TT+15) |
(Source: Maryland State Highway Administration, 2008.)
Traffic Signal Optimization Model Development and Application – Checklist
This subsection provides a high-level synthesis combining the concepts presented above on model development and application for work zone analysis involving signal optimization tools. Please note that not all the items listed below are required for all work zone analysis.
- Determine Study Objectives – The goals should directly correlate to the work zone safety, mobility, constructability concerns, and needs of the agency. The performance objectives then determine the selection of work zone alternatives or strategies that are designed to meet the established goals.
- Identify Needed Coordination Tasks – Determine what coordination must occur between other projects, agencies, facilities, and events. This input may require additional modeling to quantify impacts, such as when work is to occur on an arterial that contains mass transit.
- Determine Study Network – Determine the limits of the study network. The network boundary should be able to accommodate queues under the worst scenario, including the work zone conditions. The network also should include potential detour routes, both signed and unsigned.
- Determine Analysis Time Period – Determine the analysis time period. If needed, create different time-of-day models, such as a.m. peak, off-peak, and p.m. peak. For longer duration work zones, it is recommended that interim analysis be performed to better reflect varying capacities, if resources and data are available. Each analysis period should be long enough to include no queue at the beginning, queue buildup, and queue dissipation at the end of the analysis period.
- Confirm Analysis Method – Verify that the analysis tool(s) selected have sufficient capabilities to satisfy the analysis needs with regards to work zone characteristics, transportation management plan, data, resources, and performance measures.
- Select Measures of Effectiveness and Thresholds – The MOEs or thresholds selected will be customized to the agency’s goals and project characteristics. The MOEs and thresholds set a minimum performance standard that the alternatives must meet or exceed.
- Collect and Analyze Data – Collect data that is needed to model work zone operations. Depending on the complexity of the study network and of the analysis needed, different types of data may be required. Data may broadly be classified into four categories: general, geometric, traffic, and construction.
- Identify Work Zone Alternatives – Identify potential work zone alternatives for evaluation.
- Conduct Fatal Flaw Analysis – Perform a fatal flaw analysis on each selected alternative to determine the viability of the alternative for further analysis.
- Determine Work Zone Capacity – Roadway capacity in a work zone is reduced due to a variety of factors. It is strongly recommended that local data at work zones are collected and used. Check with the appropriate stakeholder agencies as some of them may have their own guidelines on work zone capacity and related defaults. In the absence of better local data, the analyst may use the proposed defaults as the starting point and make modifications accordingly to reflect local work zone conditions.
- Develop Existing Conditions Model – Develop an existing conditions model with the correct geometry, traffic demands, capacities, and traffic controls. Error checking should be performed to identify and correct the model coding errors.
- Calibrate Existing Conditions Model – Calibrate the model by adjusting a set of user-adjustable parameters in the analysis tool to better match specific local conditions.
- Develop Work Zone Base Conditions Model – Develop a work zone base conditions model by modifying the calibrated existing conditions model to represent work zone conditions.
- Calibrate Work Zone Base Conditions Model – Work zone model calibration includes the calibration of both work zone capacity and performance measures.
- Conduct Alternative Analysis – The alternatives analysis may involve the forecasting of the future demand and the testing of various project alternatives against the base case. The analyst needs to run the model for each alternative, review the output, compare against any performance requirements, extract relevant statistics, and perform various analyses of the results.
- Recommend Best Alternative – Utilizing a decision framework, the best overall alternative is selected.
- Document Findings – The analytical results are summarized in a final report and the analytical approaches are documented in a technical document.
4.5 Using Macroscopic Analysis
Macroscopic simulation models are based on the deterministic relationships of the flow, speed, and density of the traffic stream. The simulation in a macroscopic model takes place on a section-by-section basis, meaning they treat traffic flows as an aggregated quantity; they do not model the movement of individual vehicles on a network.
Similar to travel demand models, a key feature of macroscopic models is their ability to model large geographic areas. This ability is particularly useful when the work zone impacts may affect a larger corridor or region, where large geographic impacts need to be better understood based upon a certain work zone design such as a full closure. Macroscopic simulation models also can be set up and run fairly quickly due to the fact that they simulate aggregate flows, speeds, and density measures on each section of the network (rather than individual vehicles).
However, the simple representation of traffic movement (e.g., no car-following/lane-changing algorithms) in macroscopic simulation models may limit the fidelity of the results. Also, macroscopic simulation models may provide limited detail in simulating real-world traffic conditions. They do not have the ability to analyze transportation improvements in as much detail as the mesoscopic and microscopic models.
Some examples of macroscopic models are listed below. For a complete list, please refer to Traffic Analysis Toolbox Volume I: Traffic Analysis Tools Primer. (Alexiadis, V., K. Jeannotte, and A. Chandra. Traffic Analysis Toolbox Volume I: Traffic Analysis Tools Primer. Publication FHWA-HRT-04-038, Federal Highway Administration, U.S. Department of Transportation, Washington, D.C., June 2004.)
Data Collection
The precise input data required by a macroscopic model will vary by software and the specific modeling application as defined by the study objectives and scope. Most macroscopic studies require the following types of input data besides the general data identified at the beginning of this chapter.
Existing Models
Check if a local agency maintains a database of macroscopic model files. A field visit may need to be performed to verify the accuracy of the model input data.
Travel Demand
Most macroscopic models require O-D tables as input data. Procedures exist in many demand models to generate O-D tables for the study area. Certain macroscopic models, such as FREQ, have the capability to derive O-D tables based on traffic counts.
Calibration Data
Calibration data consist of measures of traffic counts and system performance such as travel times, speeds, delays, and queues.
Other Data
Some macroscopic models (e.g., KRONOS) require a flow-speed-density (q-v-k) relationship for each roadway type (e.g., freeway and arterial). The q-v-k relationship can be acquired using typical tube counter data, in which the average speed and counts are reported per user-defined interval. First, the counts can be converted to flow rates (q). If the reported speed is the space-mean-speed (total distance divided by total time that the speed trap is occupied), then the density can be calculated by taking k = q/v. If the average is the time-mean-speed (e.g., the average speed is the arithmetic mean of the individual speed measures), then three percent to five percent reduction from the time-mean-speed can be used to approximate the space-mean-speed.
Capacity in Modeling Tools
At the beginning of this chapter, details on work zone lane capacity were presented. It is strongly recommended that local data at work zones be collected and used, as driver characteristics vary from region to region and capacity may vary from location to location. In the absence of better local data, the analyst may use the capacity defaults as the starting point, and make modifications accordingly to reflect local work zone conditions.
Capacity can be directly specified in most macroscopic models. Typically, the user can adjust the capacity for any type of link in the network, if needed. In addition, the jam density and speed limit (if appropriate) can be modified as well for capacity calibration. Table 33 lists major parameters that the analyst may adjust for several commonly used macroscopic simulation software.
Table 33. Parameters to Adjust for Work Zone Analysis in Common Macroscopic Simulation Software
Signal Optimization Software |
Primary Parameters |
Secondary Parameters |
FREQ |
- Capacity
- Speed
- Speed curves (volume-speed relationship)
|
- Traffic composition (percent trucks)
|
KRONOS |
- Capacity
- Minimum delay speed
- Jam density
- Critical density
|
- Traffic composition (vehicle types)
|
NetZone |
- Capacity
- Capacity reduction (Percent)
- Free-flow speed
- Jam density
- Critical density
- Queue storage (vehicle/mile)
|
- Pre-trip traveler information factor
|
Key Considerations Regarding Use for Analysis
The strength of macroscopic simulation models is their ability to model large geographic areas. This ability is particularly useful when the work zone impacts may affect a larger corridor or region. Macroscopic simulation models can be set up and run fairly quickly due to the fact that they simulate aggregate flows, rather than individual vehicles.
Discussed below is the model development and application process when using macroscopic simulation tools.
Model Development and Application Process
The typical model development and application process, which has been discussed at the beginning of this chapter, applies to macroscopic simulation as well.
The calibration of macroscopic simulation models typically involves replication of observed congestion patterns. Freeway validation is based on travel time run information and speed contour diagrams constructed for the analysis periods, which are then aggregated to provide a “typical” congestion pattern. Surface-street calibration is based on speed, queue, delay, and capacity information. Macroscopic models have considerably less demanding computer requirements than microscopic models. They do not, however, have the ability to analyze transportation improvements in as much detail as mesoscopic or microscopic models; and do not consider trip generation, trip distribution, and mode choice in their evaluation of changes in transportation systems.
Macroscopic Simulation Model Development and Application – Checklist
This subsection provides a high-level synthesis combining the concepts presented above on model development and application for macroscopic tool work zone analysis. Please note that not all the items listed below are required for all work zone analysis.
- Determine Study Objectives – The goals should directly correlate to the work zone safety, mobility, constructability concerns, and needs of the agency. The performance objectives then determine the selection of work zone alternatives or strategies that are designed to meet the established goals.
- Identify Needed Coordination Tasks – Determine what coordination must occur between other projects, agencies, facilities, and events. This input may require additional modeling to quantify impacts, such as when work is to occur on an arterial that contains mass transit.
- Determine Study Network – Determine the limits of the study network. The network boundary should be able to accommodate queues under the worst scenario, including the work zone conditions. The network also should include potential detour routes, both signed and unsigned.
- Determine Analysis Time Period – Determine the analysis time period. If needed, create different time-of-day models, such as a.m. peak, off-peak, and p.m. peak. For longer duration work zones, it is recommended that interim analysis be performed to better reflect varying capacities, if resources and data are available. Each analysis period should be long enough to include no queue at the beginning, queue buildup, and queue dissipation at the end of the analysis period.
- Confirm Analysis Method – Verify that the analysis tool(s) selected have sufficient capabilities to satisfy the analysis needs with regards to work zone characteristics, transportation management plan, data, resources, and performance measures.
- Select MOEs and Thresholds – The MOEs or thresholds selected will be customized to the agency’s goals and project characteristics. The MOEs and thresholds set a minimum performance standard that the alternatives must meet or exceed.
- Collect and Analyze Data – Collect data that is needed to model work zone operations. Depending on the complexity of the study network and of the analysis needed, different types of data may be required. Data may broadly be classified into four categories: general, geometric, traffic, and construction.
- Identify Work Zone Alternatives – Identify potential work zone alternatives for evaluation.
- Conduct Fatal Flaw Analysis – Perform a fatal flaw analysis on each selected alternative to determine the viability of the alternative for further analysis.
- Determine Work Zone Capacity – Roadway capacity in a work zone is reduced due to a variety of factors. It is strongly recommended that local data at work zones be collected and used. Check with the appropriate stakeholder agencies as some of them may have their own guidelines on work zone capacity and related defaults. In the absence of better local data, the analyst may use the proposed defaults as the starting point and make modifications accordingly to reflect local work zone conditions.
- Develop Existing Conditions Model – Develop an existing conditions model with the correct geometry, traffic demands, capacities, and traffic controls. Error checking should be performed to identify and correct the model coding errors.
- Calibrate Existing Conditions Model – Calibrate the model by adjusting a set of user-adjustable parameters in the analysis tool to better match specific local conditions.
- Develop Work Zone Base Conditions Model – Develop a work zone base conditions model by modifying the calibrated existing conditions model to represent work zone conditions.
- Calibrate Work Zone Base Conditions Model – Work zone model calibration includes the calibration of both work zone capacity and performance measures.
- Conduct Alternative Analysis – The alternatives analysis may involve the forecasting of the future demand and the testing of various project alternatives against the base case. The analyst needs to run the model for each alternative, review the output, compare against any performance requirements, extract relevant statistics, and perform various analyses of the results.
- Recommend Best Alternative – Utilizing a decision framework, the best overall alternative is selected.
- Document Findings – The analytical results are summarized in a final report and the analytical approaches are documented in a technical document.
4.6 Using Mesoscopic Analysis
As discussed above, macroscopic models can only provide limited detail in simulating real-world traffic conditions. In certain cases, more in-depth simulation results are required. This is where mesoscopic models come into play.
Mesoscopic simulation models combine the properties of both macroscopic and microscopic simulation models (discussed in the next section). As in microscopic models, the mesoscopic model’s unit of traffic flow is the individual vehicle. Their movement, however, follows the macroscopic properties of traffic flows (such as average speed, density, and flow rates) without having to examine the interaction of individual vehicles in detail. Therefore, the mesoscopic model provides greater computational efficiency that allows a much faster simulation of larger networks than microscopic models, while providing users with more detailed information than macroscopic models. For instance, the lane-change maneuver could be represented for individual vehicles as an instantaneous event with the decision based on relative lane densities, rather than detailed vehicle interactions. Another example is that users have the capability to model diversion routes from major roadways (e.g., freeways and highways) to other road types (e.g., signalized arterial).
In short, mesoscopic simulation strikes a balance between the macroscopic and microscopic models. Mesoscopic models provide users with higher accuracy in simulating real-world traffic behavior than macroscopic models, but less accuracy than microscopic models. Mesoscopic analysis has the ability to analyze larger geographic areas than microscopic analysis, while still providing some of the detailed data that macroscopic analysis cannot provide.
If agency policy stipulates a need to measure lane-by-lane queue lengths, the most accurate results will most likely be generated by a microscopic model, since it provides results at the vehicle and lane level. However, microscopic tools are particularly complex and costly to deploy when modeling large corridors and regions. In these cases, a mesoscopic model or sketch-planning model could be used to predict queue lengths when lane-level results are not critical. It is important to select the approach that best provides the needed measures relative to the available data, resources, and work zone attributes.
Some examples of mesoscopic traffic simulation models are listed below. For a complete list, please refer to Traffic Analysis Toolbox Volume I: Traffic Analysis Tools Primer. (Alexiadis, V., K. Jeannotte, and A. Chandra. Traffic Analysis Toolbox Volume I: Traffic Analysis Tools Primer. Publication FHWA-HRT-04-038, Federal Highway Administration, U.S. Department of Transportation, Washington, D.C., June 2004.)
Data Collection
The precise input data required by a mesoscopic model will vary by software and the specific modeling application, as defined by the study objectives and scope. Most mesoscopic analysis work zone studies require the following types of input data besides the general data identified at the beginning of this chapter.
Existing Models
Check if a local agency maintains a database of mesoscopic model files. A field visit may need to be performed to verify the accuracy of the model input data.
Travel Demand (O-D Table)
Mesoscopic models require O-D tables as input data. Procedures exist in many demand modeling software packages to generate O-D tables for the study area.
Calibration Data (Traffic Counts and Performance Data, Such As Speed and Queues)
Calibration data consist of measures of traffic counts and system performance such as travel times, speeds, delays, and queues.
In addition to the above basic input data, speed and density data for each roadway type (e.g., freeway and arterial) are required as most mesoscopic models move vehicles according to the speed-density (v-k) relationship. The v-k relationship can be acquired using typical tube counter data in which the average speed and counts are reported per user-defined interval. First, the counts can be converted to flow rates (q). If the reported speed is the space-mean-speed (total distance divided by total time that the speed trap is occupied), then the density can be calculated by taking k = q/v. If the average is the time-mean-speed (e.g., the average speed is the arithmetic mean of the individual speed measures), then three percent to five percent reduction from the time-mean-speed can be used to approximate the space-mean-speed.
Capacity in Modeling Tools
At the beginning of this chapter, details on work zone lane capacity were presented. It is strongly recommended that local data at work zones be collected and used, as driver characteristics vary from region to region and capacity may vary from location to location. In the absence of better local data, the analyst may use the capacity defaults as the starting point and make modifications accordingly to reflect local work zone conditions.
Capacity can be directly specified in most mesoscopic models. Typically, the user can adjust the capacity for any link in the network, if needed. In addition, the jam density and speed limit (if appropriate) can be modified as well for capacity calibration. Table 34 lists major parameters that the analyst may adjust for several commonly used mesoscopic simulation models.
Table 34. Parameters to Adjust for Work Zone Analysis in Common Mesoscopic Simulation Software
Mesoscopic Simulation Software |
Parameters |
AIMSUN/Meso |
- Maximum speed
- Jam density
- Curvature geometries
- Intersection geometries
- Reaction time factor
- Look ahead distance
|
DynaMIT/DYNASMART |
- Density
- Saturation flow rate
- Maximum service flow rate
- Travel/route guidance
- Weaving sections
|
DYNAMEQ |
- Free-flow speed
- Mandatory lane changes
- VMS
|
DynusT |
- Service flow rate
- Saturation flow rate
- Jam density
- Speed limit
|
TRANSMODELER/Meso |
- Lane width factor
- Speed limit
- Saturation flow rates
- Look ahead
|
Key Considerations Regarding Use for Analysis
The strength of mesoscopic simulation models is the ability to model both large geographic areas and corridors. In addition, diversion routes and signalized intersections can be modeled. In the case of a corridor with a freeway and a signalized arterial road running through it, the diversion route onto the arterial could be more readily modeled using a mesoscopic simulation model. Mesoscopic traffic simulation models can provide knowledge of route choice behavior and are ideal for prediction applications, where the detailed modeling of route choice and other strategic driver choices are essential, but where the detailed modeling of driver interaction with the road network and other drivers is not needed.
Mesoscopic simulation models are useful for simulating existing and work zone traffic conditions for complex work zone scenarios. Discussed below are key considerations when using mesoscopic simulation tools, including:
- DTA;
- Network convergence; and
- Model development and application process.
Dynamic Traffic Assignment (DTA)
DTA models are well suited for work zone analysis due to their capability of assigning traffic dynamically. (A Primer for Dynamic Traffic Assignment. ADB30 Transportation Network Modeling Committee, Transportation Research Board, 2010.) DTA models are more responsive to operational factors (e.g., queuing) than traditional travel demand models. Also, DTA models can dynamically assign traffic over a much larger network than microscopic models. It helps to assess impacts of different work zone management strategies at the network level, not just at the corridor level.
DTA models have become a viable modeling option recently. DTA models supplement existing travel demand models and microscopic simulation models. Travel demand models represent the static regional travel demand analysis capability, whereas microscopic simulation models are superior for dynamic travel analysis at the corridor level. DTA models fill in the gap by enabling dynamic traffic to be modeled at a range of scales from the corridor level to the regional with expanded and unique functional capabilities enabled by the DTA methodology.
The static traffic assignment used in travel demand models determines network traffic flows and conditions resulting from demand/supply interactions via route choices from travelers. Route choice behavior is based upon the assumption that all travelers are aimed at traveling from their origin to their destination with the least travel time. When each traveler succeeds in finding such a route, every used route has the minimum time or cost between its origin and destination; moreover, for each O-D pair, every route used has the same travel time. This condition is known as user equilibrium. Therefore, in travel demand models, trips are instantaneously on all links between origins to destinations (not time-dependent). In addition, static traffic assignment does not take into consideration congestion spillback (i.e., where congested traffic spans a sequence of two or more links due to downstream bottleneck).
Unlike the static traffic assignment, DTA considers time variations in traffic flows and conditions, thus, attempting to reflect the reality of that traffic network. To represent these time-varying conditions in the context of user equilibrium, two concepts must be recognized: 1) in a dynamic approach, the user equilibrium condition of equal travel times on used routes applies only to travelers who are assumed to depart at the same time between the same O-D pair, which means the equilibrium condition is disaggregated to consecutive departure times instead of the entire analysis period; and 2) travel times on network links vary over time based on preceding experienced travel times, as this will depend on when travelers arrive at the various links along a route.
DTA models seek to provide a more detailed means to represent the interaction between travel choices, traffic flows, and time and cost measures in a temporally coherent manner (e.g., further improve upon the existing time-of-day static assignment approach). More specifically, DTA models aim to describe such time-varying network and demand interaction using a behaviorally sound approach. The DTA model analysis results can be used to evaluate more meaningful performance measures related to individual travel time and cost, as well as systemwide network measures for regional planning purposes.
DTA in Microscopic Simulation Models
Currently, many microscopic models are route-based, meaning vehicles select a route at departure and follow that route with or without further update along the journey during simulation. The “one-shot” (non-iterative) assignment-simulation approach is commonly used in microscopic simulation models, in which vehicles departing at different times are given a route that is periodically updated in simulation based on instantaneous travel times, not experienced travel time.
- Instantaneous Travel Time – It is a snapshot travel time measured at the time that the routes are generated without considering congestion during subsequent time periods. Such an assignment can be regarded as if travelers strictly follow some type of “pre-trip” route guidance.
- Experienced Travel Time – Experienced travel time is used in DTA models instead. The experienced travel time needs to be evaluated “after the fact,” at which point the traffic condition along the entire journey is revealed and experienced. In other words, choosing a minimal experienced travel time route at departure involves anticipation of future traffic condition along the journey. This anticipation is usually formed by learning from prior experience (e.g., try different routes). To account for this “learning” process, an iterative algorithmic process is needed. Such an iterative process reflects the learning and adjustment in route choice from one iteration to the next until the traveler cannot find a route with a shorter experienced travel time. In reality, the majority of travelers choose a route that leads to the minimal experienced travel time instead of minimal instantaneous travel time.
The difference between experienced and instantaneous travel times can be better illustrated by using Figure 14, which is a simple one-way network with four nodes and three links. (A Primer for Dynamic Traffic Assignment. ADB30 Transportation Network Modeling Committee, Transportation Research Board, 2010.) The stack of values represents the different times to traverse a link when departing from the upstream node (and entering the link) at different times. Time-varying link travel time is common during peak hours due to congestion buildups. As an example, the time needed to traverse link 1 is 1 time unit when departing the upstream node at time 1, and 3 time units when departing the upstream node at time 5. Similarly, the travel time for link 2 is 1 and 4 time units when departing the link 2 upstream node at times 1 and 5, respectively.
- The instantaneous travel time for the entire route at each different departure time is calculated by summing up the link travel time corresponding to that same departure time for all links comprising the route. As an example, for vehicles departing at time 1, the travel time is 1+1+1=3 time units; for vehicle departing at time 2, the travel time is 1+2+3=6 time units.
- The experienced travel time calculation accounts for the time needed for traversing one link, and looks up the downstream link travel time based on the time of entering that downstream link (assuming that traversing a node takes no time). Based on this approach, the travel time for the route when starting at departure time 1 should be 1 + 2 (vehicle entering link 2 at time 2, so the link 2 travel time is 2 time units), plus 6 (vehicle arriving at link 3 at time 4, so the link 3 travel time is 6 time units). The experienced travel time is 1+2+6=9 time units.
Clearly, these two methods produce different route travel times and, likewise, differing results for the shortest routes. The shortest route obtained based on the instantaneous travel time calculation has the minimum travel time based on the “snapshot” of the link travel times prevailing at departure. However, because link travel times change dynamically (due to congestion), that route does not necessarily result in minimal experienced travel time, because there is no provision in this procedure to reflect the anticipation of congestion that is to occur at a later time down the road (e.g., congestion caused by vehicles departing later but entering the same link, one which the vehicle being modeled is still traversing).
Assigning vehicles with an instantaneous travel time route is not necessarily “incorrect.” The route choice associated with instantaneous travel time may be interpreted as: 1) travelers know what the shortest route is at departure through pre-trip information (e.g., 511, news or web site) or en-route in-vehicle information system (if the traveler is to take another route when en-route); or 2) from day to day, travelers do not assess the route travel time from the experience standpoint, but rely instead on the traveler information. In contrast, the shortest route obtained based on the experienced travel time calculation method will yield a time-dependent shortest route with minimal experienced travel time. This assumes that travelers are willing to seek routes that minimize their experienced travel time instead of the route that appears to be the best only at the departure.
Figure 14. Experienced Travel Time versus Instantaneous Travel Time
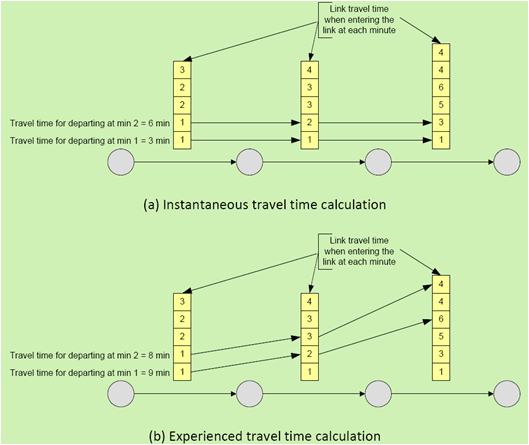
(Source: Transportation Research Board, 2010.)
Network Convergence
Almost all DTA models apply an iterative algorithm to compute an approximation to dynamic user equilibrium. From one iteration to the next, time-dependent route choices and link flows and times change as the algorithm progresses towards an equilibrium solution. At some point, the convergence criteria are satisfied and the algorithm terminates.
A poorly converged network may have the problem of “nonlocal impacts” – a minor change in the network has large impacts on flows or conditions far from the location of the change. For example, a minor change, such as a speed limit change on a particular link, would not be expected to significantly affect flows and conditions far from the link in question. DTA model outputs that showed such effects should be closely examined. When an apparent nonlocal impact exists, it is best to verify the quality of the computed convergence solution, for example, by resolving the DTA model with more stringent convergence criteria.
However, stricter convergence criteria lead to increased computational time. Therefore, a tradeoff between convergence and computational time must be made when choosing the appropriate convergence criteria.
Model Development and Application Process
The typical model development and application process, which was discussed at the beginning of this chapter, applies to mesoscopic models as well.
- For mesoscopic models, typically two forms of calibration are required; they are:
- Capacity Calibration – Factors that influence capacity calibration typically include maximum speeds, jam densities, number of lanes, curvature geometries, intersection geometries, and other minor characteristics.
- O-D Matrix Calibration – This is a more time-consuming effort as numerous iterations are often required to produce an O-D matrix that results in realistically modeled traffic. This involves utilizing an existing demand matrix and then make some adjustments to refine the accuracy of simulated flow compared with observed traffic flow.
- The O-D matrix calibration process is illustrated in Figure 15. The mesoscopic model is run with the estimated time-dependent O-D matrices. With the difference between the simulated link counts and the field counts, an optimization tool is typically used to calibrate the O-D matrices, resulting in new O-D matrices. The iterations continue until the total deviation between the simulated and field counts is less than a user-defined threshold, or when the maximum number of iterations is reached.
Figure 15. O-D Matrix Calibration in Mesoscopic Models
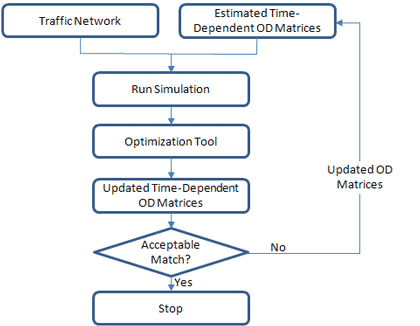
Mesoscopic Simulation Model Development and Application – Checklist
This subsection provides a high-level synthesis combining the concepts presented above on model development and application for mesoscopic simulation work zone analysis. Please note that not all the items listed below are required for all work zone analysis.
- Determine Study Objectives – The goals should directly correlate to the work zone safety, mobility, constructability concerns, and needs of the agency. The performance objectives then determine the selection of work zone alternatives or strategies that are designed to meet the established goals.
- Identify Needed Coordination Tasks – Determine what coordination must occur between other projects, agencies, facilities, and events. This input may require additional modeling to quantify impacts, such as when work is to occur on an arterial that contains mass transit.
- Determine Study Network – Determine the limits of the study network. The network boundary should be able to accommodate queues under the worst scenario, including the work zone conditions. The network also should include potential detour routes, both signed and unsigned.
- Determine Analysis Time Period – Determine the analysis time period. If needed, create different time-of-day models, such as a.m. peak, off-peak, and p.m. peak. For longer duration work zones, it is recommended that interim analysis be performed to better reflect varying capacities, if resources and data are available. Each analysis period should be long enough to include no queue at the beginning, queue buildup, and queue dissipation at the end of the analysis period.
- Confirm Analysis Method – Verify that the analysis tool(s) selected have sufficient capabilities to satisfy the analysis needs with regards to work zone characteristics, transportation management plan, data, resources, and performance measures.
- Select MOEs and Thresholds – The MOEs or thresholds selected should be customized to the agency’s goals and project characteristics. The MOEs and thresholds set a minimum performance standard that the alternatives must meet or exceed.
- Collect and Analyze Data – Collect data that is needed to model work zone operations. Depending on the complexity of the study network and of the analysis needed, different types of data may be required. Data may broadly be classified into four categories: general, geometric, traffic, and construction.
- Identify Work Zone Alternatives – Identify potential work zone alternatives for evaluation.
- Conduct Fatal Flaw Analysis – Perform a fatal flaw analysis on each selected alternative to determine the viability of the alternative for further analysis.
- Determine Work Zone Capacity – Roadway capacity in a work zone is reduced due to a variety of factors. It is strongly recommended that local data at work zones be collected and used. Check with the appropriate stakeholder agencies as some of them may have their own guidelines on work zone capacity and related defaults. In the absence of better local data, the analyst may use the proposed defaults as the starting point and make modifications accordingly to reflect local work zone conditions.
- Develop Existing Conditions Model – Develop an existing conditions model with the correct geometry, traffic demands, capacities, and traffic controls. Error checking should be performed to identify and correct the model coding errors.
- Calibrate Existing Conditions Model – Calibrate the model by adjusting a set of user-adjustable parameters in the analysis tool to better match specific local conditions. For mesoscopic models, typically two forms of calibration are required, including capacity calibration and O-D matrix calibration.
- Verify Network Convergence – The majority of mesoscopic simulation tools use DTA to compute an approximation to dynamic user equilibrium. Once done, verify the quality of the computed convergence solution to see if it meets the appropriate convergence criteria.
- Develop Work Zone Base Conditions Model – Develop a work zone base conditions model by modifying the calibrated existing conditions model to represent work zone conditions.
- Calibrate Work Zone Base Conditions Model – Work zone model calibration includes the calibration of both work zone capacity and performance measures.
- Conduct Alternative Analysis – The alternatives analysis may involve the forecasting of the future demand and the testing of various project alternatives against the base case. The analyst needs to run the model for each alternative, review the output, compare against any performance requirements, extract relevant statistics, and perform various analyses of the results.
- Recommend Best Alternative – Utilizing a decision framework, the best overall alternative is selected.
- Document Findings – The analytical results are summarized in a final report and the analytical approaches are documented in a technical document.
4.7 Using Microscopic Analysis
Microscopic simulation tools simulate the movement of individual vehicles based on car-following and lane-changing models. Vehicles enter a transportation network using a statistical distribution of arrivals and are tracked through the network over brief time intervals (e.g., one second or a fraction of a second).
Microscopic simulation models are extensively used in a wide range of applications, including evacuation planning and work zone impact analysis. They are effective in evaluating a wide range of scenarios, including heavily congested conditions, complex geometric configurations, and system-level impacts of proposed transportation improvements that are beyond the limitations of other model types. They also are useful in analyzing key bottlenecks on roadway segments and corridors where the movement of each individual vehicle needs to be represented to better understand the impact on roadway conditions. Many transportation agencies currently use microscopic models in conjunction with other analysis tools such as travel demand models and mesoscopic models to better understand the impact of roadway geometry modifications on carrying capacity and level of service.
Some examples of microscopic traffic simulation models are listed below. For a complete list, please refer to Traffic Analysis Toolbox Volume I: Traffic Analysis Tools Primer. (Alexiadis, V., K. Jeannotte, and A. Chandra. Traffic Analysis Toolbox Volume I: Traffic Analysis Tools Primer. Publication FHWA-HRT-04-038, Federal Highway Administration, U.S. Department of Transportation, Washington, D.C., June 2004.)
Level of Effort (LOE)
The Guidance on the Level of Effort Required to Conduct Traffic Analysis Using Microsimulation evaluates four case studies of microsimulation projects of various sizes and their level of effort estimates. (Cambridge Systematics, Inc. Guidance on the Level of Effort Required to Conduct Traffic Analysis Using Microsimulation. Federal Highway Administration, U.S. Department of Transportation, May 2012.) Table 35 summarizes the labor-hour estimates expected for these case studies. Additionally, Figure 16 shows the typical percentage of labor hours required for various project tasks. These examples are meant to provide analysts and modeling managers with a frame of reference on the level of effort required to complete a microsimulation analysis. These estimates serve as a point of reference, not as absolute estimates that should be applied to all projects.
Table 35. Level of Effort for Various Microsimulation Projects
Task |
Model Size and Hours |
Small |
Medium 1 |
Medium 2 |
Large |
Data Collection |
40 |
262 |
80 |
2,000 |
Base Model and Calibration |
140 |
597 |
560 |
2,500 |
Alternatives Analysis |
280 |
1,056 |
640 |
2,520 |
Documentation/Presentations |
40 |
62 |
240 |
1,850 |
Project Management |
40 |
84 |
80 |
1,210 |
Total |
540 |
2,061 |
1,600 |
10,080 |
(Source: Cambridge Systematics, Inc., 2012.)
Figure 16. Expected Level of Effort by Project Tasks for Microsimulation Projects
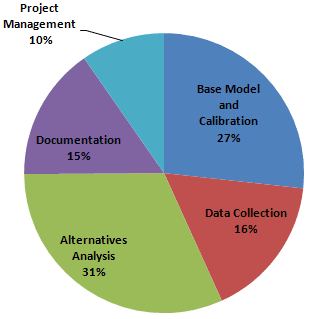
(Source: Cambridge Systematics, Inc., 2012.)
Data Collection
The precise input data required by a microsimulation model will vary by software and the specific modeling application as defined by the study objectives and scope. Most microsimulation studies require the following types of input data besides the general data identified at the beginning of this chapter.
Existing Models
Check if the local agency maintains a database of microsimulation model files. A field visit may need to be performed to verify the accuracy of the model input data.
Demands (Entry Volumes, Turning Volumes, O-D Table)
The basic travel demand data required for most models consist of entry volumes (traffic entering the study area) and traffic at ramps within the study area. Some models require one or more vehicular O-D table(s), which enable the modeling of route diversions. Procedures exist in many demand modeling software and some microsimulation software for estimating O-D tables from traffic counts.
Calibration Data (Traffic Counts and Performance Data, such as Speed and Queues)
Calibration data consist of measures of capacity, traffic counts, and measures of system performance such as travel times, speeds, delays, and queues.
Other Data
In addition to the above basic input data, microsimulation models also require data on vehicle and driver characteristics (vehicle length, maximum acceleration rate, driver aggressiveness, etc.). Because these data can be difficult to measure in the field, it is often supplied with the software in the form of various default values.
Each microsimulation model also will require various control parameters that specify how the model conducts the simulation. The user’s guide for the specific simulation software should be consulted for a complete list of input requirements.
Capacity in Modeling Tools
At the beginning of this chapter, details on work zone lane capacity were presented. It is strongly recommended that local data at work zones be collected and used, as driver characteristics vary from region to region and capacity may vary from location to location. In the absence of better local data, the analyst may use the defaults as the starting point and make modifications accordingly to reflect local work zone conditions.
Once the work zone capacity is determined, the next step is to implement it in the microsimulation model. For microsimulation tools, however, there is no direct input for “capacity.” Instead, each microsimulation software program has its own set of parameters that affect capacity, depending on the specific car-following and lane-changing logic implemented in the software. Table 36 lists major parameters that the analyst may adjust for several commonly used microsimulation software programs.
Similar to the input, there is no direct output in microsimulation models for “capacity.” Instead, the number of vehicles that pass a given point can be generated. Thus, the analyst must manipulate the input traffic demand as necessary to create a queue upstream of the target section to be calibrated so that the model will report the maximum flow rate (i.e., capacity) through the area. After the capacity is calibrated, the analyst will change the input traffic demand back.
Table 36. Parameters to Adjust for Work Zone Analysis in Common Microsimulation Software
Microsimulation Software |
Parameters |
Speed |
Car-Following |
Lane-Changing
(Before Approaching Work Zone) |
AIMSUN |
- Maximum speed
- Turn speed at intersections
- Lane speed limit
|
- Reaction time factor
- Minimum headways
|
- Maximum delay time to remove vehicles
- Look ahead distance
- Lane-changing model
- Lane selection model
- Global reaction time and local reaction time factor
|
CORSIM |
|
- Car-following sensitivity
- Headway factor by vehicle type
- Rubbernecking factor
|
- Time to complete lane-change maneuver
- Percentage of drivers yielding the right-of-way to lane-changing vehicles
- Gap acceptance
|
PARAMICS |
|
- Headway factor
- Reaction factor
|
|
VISSIM |
- Reduced speed area
- Desired speed decision
|
- Standstill distance (CC0)
- Headway time (CC1)
- Following variation (CC2)
|
- Look-back distance
- Safety distance reduction factor
- Waiting time before diffusion
- Maximum deceleration
|
TRANSMODELER |
- Lane width factor
- Tunnel factor
- Max speed difference
- Speed limit
|
- Desired speed model
- Headway thresholds
|
- Look ahead
- Critical distance
|
Key Considerations Regarding Use for Analysis
Microscopic simulation models are useful for simulating existing and work zone traffic conditions for complex work zone scenarios. Discussed below are key considerations when using microscopic simulation tools, including:
- Identifying the chokepoint;
- Work zone taper simulation;
- Model development and application process;
- Congested versus noncongested conditions;
- Initialization period;
- Number of runs; and
- Avoiding bias in the results.
Identifying the Chokepoint
For most work zones, the chokepoint will be the taper. (Horowitz, A. Microsimulation of Freeway Work Zones to Assess Flow and Capacity. University of Wisconsin-Milwaukee, Milwaukee, Wisconsin, 2009.) However, if there is an on-ramp near the taper, either upstream or downstream, the chokepoint could be within the on-ramp merge area. If the chokepoint is in an on-ramp merge area, specific care must be exercised in setting or proportioning on-ramp flow rates. Being able to identify a single chokepoint can simplify the analysis.
Work Zone Taper Simulation
A work zone taper is typically modeled as a “lane drop” in most microsimulation tools. A model can vary the amount of space ahead that drivers have to react to a lane drop, but the physical length of the taper cannot be specifically entered as a variable.
Model Development and Application Process
The typical model development and application process discussed at the beginning of this chapter applies to microscopic models as well.
Each microsimulation software package has a set of user-adjustable parameters that enable the analyst to calibrate the software to better match specific local conditions. For details on the microsimulation model calibration process, please refer to the Traffic Analysis Toolbox Volume III: Guidelines for Applying Traffic Microsimulation Software. (Dowling, R., A. Skabardonis, and V. Alexiadis. Traffic Analysis Toolbox Volume III: Guidelines for Applying Traffic Microsimulation Software. Publication FHWA-HRT-04-040, Federal Highway Administration, U.S. Department of Transportation, Washington, D.C., June 2004.)
Congested versus Noncongested Conditions
The calibration of a congested network follows the same procedure as that of noncongested ones. However, the analyst may need to pay special attention to the following issues:
- Network Boundary – Ideally, the calibrated existing conditions model (preconstruction, or “no-build”) should have its network boundary established to accommodate queues even under the worst scenario, including the work zone conditions. However, this may not be always the case. Thus, under the congested conditions, the analyst may need to extend the network further upstream so that the ends of all queues are within the simulation network.
- Temporal Boundary – The analyst also may consider expanding the temporal boundaries (i.e., the duration of the simulated time period), so that at the beginning and the end of the simulation, there are no significant queues within the network.
- Merging/Weaving Segment – Because of the lack of congestion, the microsimulation model under the noncongested conditions may be calibrated using the default driving parameters on merging/weaving segments. However, under-congested conditions, the segments with heavy merging or weaving activities may need special attention. Local lane-changing behavior parameters may need to be adjusted to reflect realistic driving conditions in the field.
Initialization Period
Simulation model runs usually start with zero vehicles on the network. If the simulation output is being compared to field measurements (as in calibration), the artificial period where the simulation model starts out with zero vehicles (the warm-up period) must be excluded from the reported statistics for system performance. Some software programs will do this automatically. For others, the warm-up period must be computed offline by the analyst. For details on how to identify the warm-up period, please refer to the Traffic Analysis Toolbox Volume III: Guidelines for Applying Traffic Microsimulation Software. (Dowling, R., A. Skabardonis, and V. Alexiadis. Traffic Analysis Toolbox Volume III: Guidelines for Applying Traffic Microsimulation Software. Publication FHWA-HRT-04-040, Federal Highway Administration, U.S. Department of Transportation, Washington, D.C., June 2004.)
Number of Runs
Random numbers are used in microsimulation models to generate vehicles, decide their behavior, and select their destination and route as they move through the network. A single simulation run cannot be expected to represent specific field conditions. It is necessary to run the model several times with different random number seeds to get the necessary output to determine mean, minimum, maximum values, and standard deviation. The multiple runs then need to be post-processed to obtain the necessary output statistics.
The determination of the number of simulation runs is an iterative process. For more information, please refer to the Traffic Analysis Toolbox Volume III: Guidelines for Applying Traffic Microsimulation Software. (Dowling, R., A. Skabardonis, and V. Alexiadis. Traffic Analysis Toolbox Volume III: Guidelines for Applying Traffic Microsimulation Software. Publication FHWA-HRT-04-040, Federal Highway Administration, U.S. Department of Transportation, Washington, D.C., June 2004.)
Avoiding Bias in the Results
The simulation geographic and temporal limits should be sufficient to include all congestion related to the base case and all of the alternatives. Otherwise, the model will not measure all of the congestion associated with an alternative, thus potentially causing the analyst to underreport the benefits of an alternative.
Microscopic Simulation Model Development and Application – Checklist
This subsection provides a high-level synthesis combining the concepts presented above on model development and application for microsimulation model work zone traffic analysis. Please note that not all the items listed below are required for all work zone analysis.
- Determine Study Objectives – The goals should directly correlate to the work zone safety, mobility, constructability concerns, and needs of the agency. The performance objectives then determine the selection of work zone alternatives or strategies that are designed to meet the established goals.
- Identify Needed Coordination Tasks – Determine what coordination must occur between other projects, agencies, facilities, and events. This input may require additional modeling to quantify impacts, such as when work is to occur on an arterial that contains mass transit.
- Determine Study Network – Determine the limits of the study network. The network boundary should be able to accommodate queues under the worst scenario, including the work zone conditions. The network also should include potential detour routes, both signed and unsigned.
- Determine Analysis Time Period – Determine the analysis time period. If needed, create different time-of-day models, such as a.m. peak, off-peak, and p.m. peak. For longer duration work zones, it is recommended that interim analysis be performed to better reflect varying capacities, if resources and data are available. Each analysis period should be long enough to include no queue at the beginning, queue buildup, and queue dissipation at the end of the analysis period.
- Confirm Analysis Method – Verify that the analysis tool(s) selected have sufficient capabilities to satisfy the analysis needs with regards to work zone characteristics, transportation management plan, data, resources, and performance measures.
- Select MOEs and Thresholds – The MOEs or thresholds selected will be customized to the agency’s goals and project characteristics. The MOEs and thresholds set a minimum performance standard that the alternatives must meet or exceed.
- Collect and Analyze Data – Collect data that is needed to model work zone operations. Depending on the complexity of the study network and of the analysis needed, different types of data may be required. Data may broadly be classified into four categories: general, geometric, traffic, and construction
- Identify Work Zone Alternatives – Identify potential work zone alternatives for evaluation.
- Conduct Fatal Flaw Analysis – Perform a fatal flaw analysis on each selected alternative to determine the viability of the alternative for further analysis.
- Determine Work Zone Capacity – Roadway capacity in a work zone is reduced due to a variety of factors. It is strongly recommended that local data at work zones be collected and used. Check with the appropriate stakeholder agencies as some of them may have their own guidelines on work zone capacity and related defaults. In the absence of better local data, the analyst may use the proposed defaults as the starting point and make modifications accordingly to reflect local work zone conditions.
- Determine Initialization Period – Simulation model runs usually start with zero vehicles on the network. Therefore, an initialization period (warm-up period), where the simulation model starts out with zero vehicles needs to be determined and used in the simulation models.
- Develop Existing Conditions Model – Develop an existing conditions model with the correct geometry, traffic demands, capacities, and traffic controls.
- Check Errors – Error checking should be performed to identify and correct the model coding errors. The error checking involves the reviews of software errors, network coding, demand data, and simulation animation.
- Calibrate Existing Conditions Model – Calibrate the model by adjusting a set of user-adjustable parameters in the analysis tool to better match specific local conditions.
- Verify Network Convergence – If dynamic assignment is used, the network convergence needs to be verified to see if it meets the appropriate convergence criteria.
- Determine the Number of Runs – A single simulation run cannot be expected to represent specific field conditions. It is necessary to run the model several times with different random number seeds to get the necessary output to determine mean, minimum, maximum values, and standard deviation.
- Develop Work Zone Base Conditions Model – Develop a work zone base conditions model by modifying the calibrated existing conditions model to represent work zone conditions.
- Simulate Work Zone Taper – A work zone taper is typically modeled as a “lane drop” in most microsimulation tools. A model can vary the amount of space ahead that drivers have to react to a lane drop, but the physical length of the taper cannot be specifically entered as a variable.
- Calibrate Work Zone Base Conditions Model – Work zone model calibration includes the calibration of both work zone capacity and performance measures.
- Conduct Alternatives Analysis – The alternatives analysis may involve the forecasting of the future demand and the testing of various project alternatives against the base case. The analyst needs to run the model for each alternative, review the output, compare against any performance requirements, extract relevant statistics, and perform various analyses of the results.
- Recommend Best Alternative – Utilizing a decision framework, the best overall alternative is selected.
- Document Findings – The analytical results are summarized in a final report and the analytical approaches are documented in a technical document.
PDF files can be viewed with the Acrobat® Reader®.