Traffic Analysis Toolbox Volume XII:
Work Zone Traffic Analysis – Applications and Decision Framework
Chapter 6. Reconciling Inconsistencies and Conducting Sensitivity Analysis
Throughout the project development process, several factors can cause variations in performance measures and outputs used to compare and analyze the benefits and impacts of work zone alternatives.
- The first section of this chapter describes the typical variations that can be expected in the analysis outputs and measures of effectiveness extracted during various stages of a work zone traffic analysis.
- The second section describes several ways to reconcile the variations and inconsistencies that can occur in modeling or analysis results.
- The third section describes how sensitivity analysis can be used to validate and rectify issues with the variations and inconsistencies resulting from the work zone modeling and analysis.
6.1 Variations in the Measures of Effectiveness, Tools, and Outputs
The project type and scale can affect the structure, the level of data collection and analysis efforts, and decision-making framework that is incorporated into developing and evaluating the project and the project development process (PDP). Each PDP stage will be driven by a different purpose and objectives, which may require different types of analysis efforts and tools. Using different tools can yield differences in output results and variations in measures of effectiveness (MOE).
Table 71 presents common measures that can be extracted from different classes of analysis tools. The table shows that although the same type of performance measures may be collected from each analysis tool, the definition of the measure and the types of outputs needed in order to calculate the MOE may differ depending on the tool used. For instance, while both signal optimization and travel demand modeling tools can report delay, only signal optimization can report intersection delay effectively.
Table 71. Performance Measures and Outputs by Tool Type
Performance Measures |
Outputs |
Tool Focus |
Sketch-Planning – Analytical/Deterministic Tools |
|
- Vehicle-Hours Traveled (VHT)
- Person-Hours Traveled (PHT)
|
Sketch-planning tools can be used for systemwide measures. Deterministic tools are better suited for facility-specific measures. |
|
- Intersection Total Delay
- Intersection Control Delay
- Stop Delay
|
Sketch-planning tools can be used for systemwide measures. Deterministic tools are better suited for facility-specific measures. |
|
- Freeway Facility Queue Density
- Percentile Probability Queues
|
Sketch-planning tools can be used for systemwide measures. Deterministic tools are better suited for facility-specific measures. |
|
- Average Speed
- Space Mean Speed
|
Sketch-planning tools can be used for systemwide measures. Deterministic tools are better suited for facility-specific measures. |
|
|
Sketch-planning tools can be used for systemwide measures. Deterministic tools are better suited for facility-specific measures. |
|
|
Sketch-planning tools can be used for systemwide measures. Deterministic tools are better suited for facility-specific measures. |
|
- For Freeway Facilities – Average Density by Segment or Across Facility
- Multilane Highways – Mean Passenger Car Density
|
Sketch-planning tools can be used for systemwide measures. Deterministic tools are better suited for facility-specific measures. |
|
|
Sketch-planning tools can be used for systemwide measures. Deterministic tools are better suited for facility-specific measures. |
Travel Demand Model Tools |
|
|
Systemwide measures. |
|
|
Systemwide measures. |
|
|
Systemwide measures. |
|
|
Systemwide measures. |
|
|
Systemwide measures. |
|
|
Systemwide measures. |
|
|
Systemwide measures. |
|
|
Systemwide measures. |
Traffic Signal Optimization Tools |
|
|
Facility-specific measures. |
|
- Total Delay
- Control Delay
- Stop Delay
- Queue Delay
|
Facility-specific measures. |
|
- Average Queue
- Maximum Queue
- 50th and 95th Percentile Queue
|
Facility-specific measures. |
|
|
Facility-specific measures. |
|
|
Facility-specific measures. |
|
- Fuel Consumption
- Fuel Economy
|
Facility-specific measures. |
|
|
Facility-specific measures. |
|
- HCM and Intersection Capacity Utilization (ICU) LOS
|
Facility-specific measures. |
Macroscopic Simulation Tools |
|
|
Facility-specific. |
|
- Total Delay
- Delay by Facility Type (i.e., Freeway, On-Ramp, and Off-Ramp)
|
Facility-specific. |
|
- Congested Length or Queue Length
|
Facility-specific. |
|
|
Facility-specific. |
|
- Volume (Expressed as Flows at an Hourly Rate)
- V/C Ratio
|
Facility-specific. |
|
|
Facility-specific. |
|
- Average Density (Vehicles per Mile per Lane)
|
Facility-specific. |
|
- LOS (Calculated as a Function of Density)
|
Facility-specific. |
Mesoscopic Simulation Tools |
|
- Travel Time, Trip Time (VHT)
|
Facility-specific. |
|
|
Facility-specific. |
|
- Entry Queues
- Vehicle Queue Length
|
Facility-specific. |
|
- System Average Speed
- Average Link Speed (mph)
|
Facility-specific. |
|
|
Facility-specific. |
|
|
Facility-specific. |
|
- Link Density (Average Passenger Car/Lane-Mile)
- Mean Passenger Car Density of Moving Vehicles
|
Facility-specific. |
|
|
Facility-specific. |
Microscopic Simulation Tools |
|
- System- and Link-Based VHT
- Total Time per Vehicle
|
Facility-specific. |
|
- Average Delay (by Link, Node, and Network-Wide)
- Delay Time or Lost Time (the Difference between the Theoretical Travel Time versus Actual Vehicle Travel Time) per Vehicle
- Delay Time Rate (Minute/Mile)
- Queue Time
- Stop Time, Stopped Delay, Average Stopped Delay
- Total Delay (Network-Wide)
|
Facility-specific. |
|
- Average Queue Length
- Maximum Queue
- Percent of Link Storage
|
Facility-specific. |
|
- Average Speed by Link
- Network-Wide Average Speed
|
Facility-specific. |
|
- Volume Count and Flow (Vehicles per Hour)
|
Facility-specific. |
|
|
Facility-specific. |
|
- Average Density per Link Segment during Analysis Time Period (Vehicles/Lane-Mile)
|
Facility-specific. |
|
- Most microsimulation tools do not report LOS. Paramics Analyzer reports HCM LOS using Passenger Car Unit (PCU) Densities
|
Facility-specific. |
Different tools also are better suited for certain levels of analysis. The levels of analysis include systemwide and facility-specific. Systemwide measures report mobility, safety, and environmental impacts for the entire system or network while facility-specific focus either on a specific link, localized area, or facility type. For instance, macroscopic models and travel demand models are best suited for systemwide measures. Due to the level of detail and complexity involved in microscopic simulation analysis, microscopic simulation tools, while capable of generating systemwide MOEs, are best utilized for reporting measures at a more localized or facility-specific level than evaluating larger systems.
The project characteristics, goals, and how the PDP is structured will affect and be impacted by the types of tools, measures, and outputs generated. The following section describes how to reconcile these issues to aid in better decision-making. It also will feature an example that depicts how variations in MOEs can occur due to the use of different analysis tools, as well as how to reconcile those inconsistencies.
Variations Based on Project Type
The types of tools utilized during a work zone traffic analysis can vary based on the characteristics of the project and the project site. Different analysis tools may yield different outputs and performance measures.
One of the major project characteristics that can impact the type of tools and MOEs used on projects include the project’s size.
- Small-scale, site-specific projects may have a more concentrated impact, a shorter construction and project development period, and potentially more limited resources. These projects may utilize sketch-planning and HCM/deterministic models, where the analysis could be done simply and quickly, with a smaller-scale data collection effort. Example measures of effectiveness (MOE) for a smaller scale project may, therefore, be at a facility level.
- Larger, systemwide projects have wider-range impacts, with longer durations, and potentially more resources. These projects, due to their size, will require tools that can handle larger networks and a range of measures that include mobility, safety, and environmental factors. The performance measures would most likely be at a systemwide level.
- The goals and objectives of the project along with the work zone characteristics also will dictate what tool may be used and consequently what types of outputs and measures can be generated. If the project’s main objectives, for instance, require that the site be maintained at a certain level of service, the type of tools and MOEs that an agency may choose will be different if the objectives focus on issues related more to safety.
Variations Based on Project Stage
Each project stage will have a different objective and timeframe for when an action or decision has to occur. Specific tools may be more applicable for certain objectives and timeframes. Therefore, different tools or combination of tools may be utilized for various stages of the project development process (PDP). The following describes the types of variations in MOEs, tools, and outputs that occurs during specific phases of the project.
- Project Planning and Scoping – At the project planning stage, agency staff and decision-makers define and prioritize the goals and objectives of the project. Therefore, at the planning stage, these evaluators will typically look at measures at a systemwide level. Tools that may be used include sketch-planning tools and travel demand models.
- Environmental, Preliminary Design/Project Approval Stage – The objectives at this stage of the project are to develop the preliminary design and operations plan, obtain environmental clearance, and receive approval to proceed with the next stage. At this stage, a preliminary design and list of potential alternatives will be evaluated for the project. The short- and long-term benefits and traffic and environmental impacts of the project also will be determined. The measures presented at this stage of the project will be targeted for decision-makers and the public. In order to determine the benefits and impacts of the project, several different tools may be used depending on the size and complexity of the project.
- For broad- and system-level performance measures, travel demand models supported by sketch-planning tools may be appropriate.
- For more complex analysis that may look at the performance of a specific facility or the performance of major highways along with adjacent arterials, a signal optimization model, mesoscopic, and/or microscopic simulation tools may be used.
- Plans, Specifications, and Estimates (PS&E), Construction, and Operation Analysis Stage:
- During the PS&E phase of the project, the agency prepares design plans and project specifications. At this stage, work zone traffic analysis is conducted in order to support the project design. The traffic analysis results ensure that the project will have the capacity to meet or service the demand for the facility.
- During construction, the agency’s objectives in conducting any modeling or analysis effort are to determine how to safely and efficiently construct a project with minimal impacts to the environment, the community, and workers. Modeling and analysis efforts for the construction stage are focused less on mobility and more towards evaluating how effective the project or work zone strategies are at improving safety and or project/construction efficiency.
- During the operations stage, agency objectives are focused on evaluating the level of service of the facility and the traffic performance of the work zone. The types of measures at this stage include mobility measures such as delay, average speed, and queue lengths. At this stage, a larger data collection effort and more complex modeling and analysis tools may be brought in.
- Alternatives Analysis – When choosing among work zone alternatives, the analyst will consider the various performance measures generated in the different project stages in order to make a decision regarding which alternative to recommend.
6.2 Achieving Consistency of Results When Using Multiple Tools
The previous section discussed the variations in measures of effectiveness, tools, and outputs that an analyst can encounter when conducting a work zone traffic analysis. These variations can cause inconsistencies in results, inaccuracies in calculating benefits and impacts, and misinformation in deciding among various alternatives. This section describes the need for consistency when using different analysis tools and reconciling the inconsistencies that may occur with different modeling outputs and measures of effectiveness. This section will provide background on consistency within the project development process, describing how inconsistencies occur and the importance of achieving consistency in outputs and measures. The section also will describe how to evaluate and ensure consistency throughout the various project stages.
Background on Consistency
“The goal of achieving or maintaining consistency throughout the project development process (PDP) ensures that the initial decision to proceed with the project is still the correct choice at the end of the process.” (Dowling, R. Guide on the Consistent Application of Traffic Analysis Tools and Methods. Publication FHWA-HRT-11-064, Federal Highway Administration, U.S. Department of Transportation, Washington, D.C., November 2011.) There are two resources that describe the potential variations and inconsistencies in MOEs and analysis results when using multiple tools. One resource is the FHWA-sponsored research, Guide on the Consistent Application of Traffic Analysis Tools, and the other is Traffic Analysis Toolbox Volume VI: Definition, Interpretation, and Calculation of Traffic Analysis Tools Measures of Effectiveness. (Dowling, R. Guide on the Consistent Application of Traffic Analysis Tools and Methods. Publication FHWA-HRT-11-064, Federal Highway Administration, U.S. Department of Transportation, Washington, D.C., November 2011. Dowling, R. Traffic Analysis Toolbox Volume VI: Definition, Interpretation, and Calculation of Traffic Analysis Tools Measures of Effectiveness. Publication FHWA-HOP-08-054, Federal Highway Administration, U.S. Department of Transportation, Washington, D.C., January 2007.) These two resources provide guidance on how different types of analysis tools produce particular MOEs and how to reconcile the potential inconsistencies that may result from using multiple tools. For further details on many of the concepts addressed in this section, please refer to these two documents.
Achieving consistency in terms of outputs, performance measures, and results is important for the following reasons:
- Consistency ensures quality and accuracy of results;
- Consistent and accurate results leads to verifiable decision-making process and outcomes;
- Consistency enhances the agency’s credibility and effectiveness;
- Consistency assures that the analysis process is effective and efficient; and
- Consistency ensures that the analysis procedures are more refined and flexible enough to be reapplied even through certain parameter changes.
As mentioned in the previous section, variations in MOEs, tools, and outputs can occur naturally depending on the project type and project development stages. When different tools and types of performance measures are used throughout one project, inconsistency in results can occur. Additionally, inconsistency may occur due to the following factors: (Dowling, R. Traffic Analysis Toolbox Volume VI: Definition, Interpretation, and Calculation of Traffic Analysis Tools Measures of Effectiveness. Publication FHWA-HOP-08-054, Federal Highway Administration, U.S. Department of Transportation, Washington, D.C., January 2007.)
- Overuse of defaults;
- Calibration mistakes;
- Inaccurate data or demand forecasts;
- Failure to check for reasonableness of results; and
- Differences in performance measure definitions across different tool types.
Reconciling Measures of Effectiveness
As discussed in Section 6.1 of this document, variations in MOEs can occur at different project stages and through the use of different analysis tools. The use of different tools can produce inconsistencies in MOE results and challenges when comparing measures across different analysis tools. In order to reconcile the potential inconsistencies that can result from the use of different tools, the analyst should keep in mind the characteristics of the tool – its strengths and limitations. For further information on the capabilities and limitations of different traffic analysis tools, refer to Chapter 3 of this document. In order to reconcile the variations in MOEs that occur from the use of different tools, the analyst should account for the following when choosing the appropriate tool:
- Choose the tool most reliable for extracting the outputs needed to generate the performance measures established.
- Choose the tool that is most appropriate for producing MOEs by the focus level (i.e., facility- or system-specific) required for the particular project stage.
- If MOEs need to be compared across tools, find the least common denominator amongst the two. According to the FHWA-sponsored Traffic Analysis Toolbox Volume VI: Definition, Interpretation, and Calculation of Traffic Analysis Tools Measures of Effectiveness, “the comparison of results between tools and methods is possible only if the analyst looks at the lowest common denominator shared by all field data collection and analytical tools.” (Dowling, R. Traffic Analysis Toolbox Volume VI: Definition, Interpretation, and Calculation of Traffic Analysis Tools Measures of Effectiveness. Publication FHWA-HOP-08-054, Federal Highway Administration, U.S. Department of Transportation, Washington, D.C., January 2007.) In this guide, vehicle trajectories are recognized as the lowest common denominator. However, the NCHRP Report 3-85-20 notes that because vehicle trajectories may be defined differently across different tools, the lowest common denominator is field observations. Because analysis tools and models should be calibrated or validated by measures from the field, field observations serve as a common link across different tools and methodologies. (The University of Florida Transportation Research Center and T-Concepts Corporation, 2009. Determination of HCM-Compatible Performance Measures from Vehicle Trajectory Analysis. NCHRP Report 3-85-20, Transportation Research Board, National Research Council, Washington, D.C., 2009.)
Reconciling Different Tools
There are several ways to reconcile the variations or inconsistencies that may be present in analysis results due to the utilization of different tools during the project development process.
- When utilizing results from different tools, the most reliable tool should always take precedence over the results from the less reliable ones. For instance during the project planning stage of an MOTAA, a travel demand model coupled with a sketch planning may be used to determine the potential work zone alternatives to be considered for a project.
- When possible the same tool should be used throughout the various project stages. For instance, if a microsimulation model was developed during the environmental clearance stage, that model can be carried over to subsequent project stages, especially during the alternatives analysis.
- While the results from the more reliable or robust tool may be used, the analyst should still review the outputs from the less reliable tools to QA/QC the preferred tool’s results. One way to do this is to use another analysis tool’s results to determine whether common trends appear in both models. For instance, when comparing the mobility impacts of a ramp closure, a traffic optimization tool may note that nearby parallel signalized arterial intersections experience a degradation of LOS from an LOS B to an LOS D. A microsimulation tool may express this degradation in mobility levels with measures such as delay, speed reduction, or queue length. Although the values and measures are different, the two tools should agree that there are congestion issues at that particular section of the project area.
- Further checks on the modeling and analysis tool results should include error checking of the model network coding, volumes, and speeds by comparing the model inputs and outputs with observed traffic statistics.
Reconciling Outputs
When different tools must be used during the project development process, inconsistencies amongst model outputs are likely to occur. The following presents various ways to reconcile these differences: (Dowling, R. Guide on the Consistent Application of Traffic Analysis Tools and Methods. Publication FHWA-HRT-11-064, Federal Highway Administration, U.S. Department of Transportation, Washington, D.C., November 2011.)
- The tool that is used to report the facility-specific performance analysis should be used to report all facility performance results. For instance, if CORSIM was used to generate facility travel times, it also should be used to identify bottlenecks, queues, speeds, and delays for the facility. This would be the same for system-specific performance measures.
- When different tools have similar outputs but are defined differently, the analyst should be able to compare and understand the differences between the tools.
- If neither tool provides the best or most complete solution to a problem, the analyst may want to consider the following:
- Additional data processing may be needed to reconcile the differences by using revised outputs that utilize a combination of the outputs from the different tools.
- It may be necessary to convert one tool’s outputs so that it can be compared at the same level as the other tool.
Reconciling Variations and Inconsistencies Example: I-15 Ontario Corridor Pavement Reconstruction Project
An example of a work zone project that used different tools throughout the various stages of the project was the I-15 Pavement Reconstruction Project in Ontario, California. This work zone project required the reconstruction of two of three truck lanes in each direction of the interstate. For further details regarding the project refer to the Traffic Analysis Tools Volume IX: Work Zone Modeling and Simulation – A Guide for Analysts. (Hardy, M., and K. Wunderlich. Traffic Analysis Toolbox Volume IX: Work Zone Modeling and Simulation – A Guide for Analysts. Publication FHWA-HOP-09-001, Federal Highway Administration, U.S. Department of Transportation, Washington, D.C., March 2009.) Figure 32 shows the analysis process for I-15 project and presents when each analysis tool was used and for which stage of the project. Table 72 shows the various tools, measures, and outputs utilized throughout various stages of the project.
Figure 32. I-15 Analysis Process
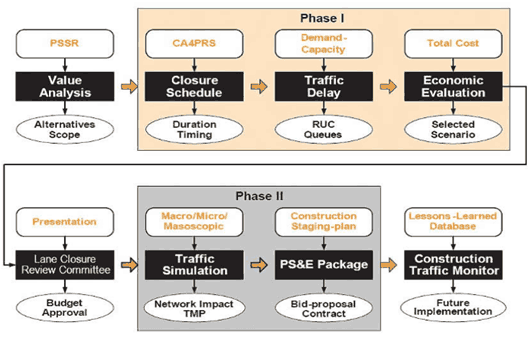
(Source: Hardy and Wunderlich, 2009.)
Table 72. MOEs, Tools, and Outputs Utilized for I-15 Pavement Reconstruction Case Study
Phase |
Stage |
MOEs |
Analysis Tool |
Outputs |
1 |
- Planning/Scoping (Preconstruction Plans)
|
|
|
- Total number of lane closures
|
- Alternatives Analysis (Preconstruction Plan)
|
- Facility-specific (delays reported for the entire corridor but only one facility type, freeway involved in analysis)
|
- HCM Demand-Capacity Model
|
- Total and max delay; road user cost
|
2 |
- PS&E and Operational Analysis
|
- Systemwide
- Facility-specific
|
|
- Staging analysis plan (number of weekends needed, working days, etc.)
- Delay in minutes (reported by segmentation defined by the six construction staging scenarios)
|
The I-15 project used three traffic modeling tools, including the Construction Analysis for Pavement Rehabilitation Strategies (CA4PRS), Highway Capacity Manual Demand-Capacity Model (HCM), and Dynameq mesoscopic traffic simulation tool. During the preconstruction planning, alternatives development, and initial alternatives analysis portion of the project, the analysts utilized CA4PRS and HCM to analyze six alternative construction scenarios. Table 73 shows the preconstruction analysis results from HCM and CA4PRS. As shown by the results, Scenario 1 was the most cost-effective and was, therefore, chosen as the preferred alternative.
Table 73. I-15 Preconstruction Analysis Results
Scenario Name |
Closure Scheme |
Closure Assessment (Number of Closures) |
Traffic Analysis |
Cost Analysis |
Total Cost
(Total Cost = (RUC/3) + Cost Analysis) |
RUC
(Million Dollars) |
Delay
(Minutes) |
Original |
Median and structure widening |
35 weekends |
3 |
16 |
78 |
79 |
Rapid Rehab 1 |
Full closure one roadbed |
35 weekends |
119 |
363 |
83 |
123 |
Rapid Rehab 2 |
Full closure one roadbed |
8 weeks |
123 |
363 |
77 |
118 |
Traditional |
Partial closure |
1,220 nights |
133 |
22 |
88 |
113 |
Long-Life CSOL |
Full closure one roadbed |
20 weekends |
69 |
363 |
60 |
83 |
The second phase of the project entailed the development of a construction staging plan and traffic management plan. The agency, therefore, had to conduct further analysis of the preferred alternative in order to develop a construction staging plan that optimized construction schedule and resources while minimizing impacts to the public. CA4PRS was used to develop the more detailed construction staging plan of the preferred alternative and mesoscopic simulation tool, Dynameq, was used to evaluate the mobility impacts (delay) associated with the staging plan options. The final six construction staging scenarios included the following:
- 2B – Closure of I-10 West to I-15 South and I-15 South to Jurupa ramps;
- 2C – Closure of I-10 East to I-15 South ramp;
- 2D – Closures of I-15 South to I-10 West and from 4th Street to I-15 South ramps;
- 3D – Closures of Jurupa to I-15 North and I-15 North to I-10 East and I-10 West ramps;
- 3F – Closure of EB I-10 to NB I-15 connector and both NB ramps at 4th Street; and
- 4B – Closure of SB I-15 to WB and EB SR 60.
The following describes how each tool was used:
- CA4PRS is a sketch-planning tool that was used in the preconstruction analysis and planning stage in order to identify rehabilitation strategies such as closure schemes that optimized construction production, schedule, and minimized inconvenience to the public. It was used in the second phase to analyze the preferred alternative and develop a more detailed staging analysis plan. CA4PRS also was chosen because results from this tool were easily integrated into traffic simulation tools for the calculation of road user costs.
- HCM Demand-Capacity Model (HCM) was used to analyze the impacts of the work zone closure strategies identified through CA4PRS. Using this model, the analysts were able to calculate total and maximum delays as well as road user costs. The California Freeway Performance Measurement System (PeMS) was used to estimate traffic demand.
- Dynameq, a mesoscopic simulation modeling tool, was used to assist in the development of the transportation management plan (TMP). Dynameq was used to evaluate the delays stemming from the six staging scenarios. It also was used because it incorporated the impacts of detour routes in generating the delay calculations.
Although different tools were utilized during the various stages of the project, the agency managed to achieve consistency by following some of the concepts stated earlier in this chapter:
- Choose the tool most reliable for extracting the outputs needed to generate the performance measures established.
- The agency needed a simple analysis using historical work zone data that could guide them towards appropriate work zone and lane closure strategies. Sketch-planning tools, specifically, CA4PRS was best used for determining appropriate work zone strategies based on data from projects with similar characteristics and particular traffic conditions and scenarios.
- Since CA4PRS did not have the appropriate modules for conducting HCM calculations at the time, the agency made the appropriate choice to use another tool, HCM demand-capacity model, order to calculate the necessary mobility measures needed to evaluate and compare the lane closure alternatives.
- In order to determine appropriate detour routes, the analysts needed the use of a traffic modeling tool that analyzed diversions and route shifts. The agency chose the appropriate tool that would generate these performance measures, mesoscopic tool Dynameq.
- Choose the tool that is most appropriate for producing MOEs by the focus level.
- One example of how the agency followed this concept is through their choice of using a mesoscopic model. Since the traffic analysis for the project was for a larger study area, it might have been appropriate to use a macroscopic model. However, because of the number of detour routes involved in the analysis, macroscopic could not be used and microscopic simulation would be too resource intensive and complex for a study area of this size. The analysts, therefore, chose the most appropriate tool, mesoscopic, for the size of the project area and the scope of the analysis.
- Further checks on the modeling and analysis tool results should include error checking of the model network coding, volumes, and speeds by comparing the model inputs and outputs with observed traffic statistics.
- PeMS was used to verify the travel demand forecasts.
6.3 Sensitivity Analysis
The previous sections discussed the potential for inconsistencies with the analysis results and described how to reconcile those differences. Because of the dynamic nature of traffic conditions, sensitivity analysis can be used for verifying the quality and accuracy of results as well as providing further insight into acceptable ranges of results based on different traffic conditions and scenarios. This section is structured to provide an introduction to sensitivity analysis and its role within a work zone traffic analysis. The subsequent parts of the section provide an overview of how and when to use sensitivity analysis during different stages of the project, such as during:
- Demand forecasting and capacity analysis;
- Model validation and calibration; and
- Alternatives analysis – decision-making and performance monitoring.
Each section describes how sensitivity analysis can be used for that particular stage. Case studies and examples explain the key concepts for applying such analysis tools and methods.
Introduction and Purpose of Sensitivity Analysis
Sensitivity analysis is a quantitative methodology that can be employed to do the following:
- Reconcile the variations in MOEs and analysis results;
- Determine the robustness of a result;
- Support recommendations or decisions;
- Identify critical values or solutions;
- Better understand the relationship between different variables, inputs, and outputs;
- Identify potential errors; and
- Improve and ensure accuracy of a model.
How a sensitivity analysis is conducted or structured can differ depending on its purpose and the resources available to conduct the analysis. There are some common steps involved in most sensitivity analysis. These steps include the following:
- Defining the objective of the sensitivity analysis – answering the question “What to test?”
- Determining which parameters to vary and which to remain constant.
- Determining what to observe.
- Determining which analysis outputs are relevant to the objective.
- Determining the design of the analysis – such as the combination of parameters, which parameters to vary, when they should change, and by how much.
Sensitivity Analysis in Demand Forecasting and Work Zone Capacity Analysis
This section provides an overview of how sensitivity analysis can be used to further support results from demand forecasting as well as work zone capacity analysis. As with most analysis tools there are limitations and gaps in a travel demand model’s capability to capture the potential impacts of work zone alternatives and mitigation strategies (i.e., travel demand management, intelligent transportation systems, and traveler information strategies), as well as the effects of certain scenarios and travel conditions (i.e., normal peak and off-peak conditions, incident, special events, and work zones).
All forecasts are subject to uncertainty, as minor changes to certain model parameters can significantly impact the demand and capacity results of a model. It also can impact the measures derived from demand and capacity such as the project’s mobility (delay, queue, and speed) impacts, benefits, and costs. Therefore, it is important to understand the potential variations in demand forecasts and capacity estimations, especially when using such to estimate other impacts and benefits.
Sensitivity analyses can be conducted to validate and support the methodologies used to estimate work zone traffic demand and capacity. It also can aid in identifying the factors that impact demand and capacity, as well as the appropriate demand and/or capacity ranges that could be expected for particular work zone projects and alternative scenarios. The following provides examples describing how sensitivity analyses can be used to support demand and capacity measures for work zones.
Demand/Capacity Scenarios – Q-DAT
In one sensitivity analysis approach, the Texas Transportation Institute (TTI) utilizes their developed tool, Q-DAT, to evaluate potential work zone alternatives by varying demand and capacity levels to determine how they impact delay and queues in the project area. In Q-DAT, the delay and queue estimation function of the tool calculates delay per vehicle and average queue length for three scenarios with varying traffic volumes and work zone capacities, “Expected Case,” “Best Case,” and “Worst Case.”
- Expected Case – In the “Expected” case, the traffic volumes and work zone capacity are based on user input. These could be based on experience, historical information, or field observations.
- Best Case – The “Best Case” reflects traffic volumes that are 10 percent lower and work zone capacity 10 percent higher than the user inputs.
- Worst Case – The “Worst Case” scenario is based on traffic volumes that are 10 percent higher and work zone capacity that is 10 percent lower than the user input.
Analyzing the variations in queue and delay impacts of these three scenarios serves as a sensitivity analysis for determining how varying demand and capacity levels impact mobility within a work zone. By evaluating a work zone project based on these three scenarios, an agency can have a wider spectrum or range of potential mobility impacts that could be expected at the work zone. Such information can be used to shape mitigation strategies as well as potential work zone alternatives (i.e., nighttime construction, partial and full lane closures, and detours) they may consider.
Variations in Traffic Demand and Capacity Parameters Due to Specific Travel Conditions
Sensitivity analyses can be used to determine appropriate demand and capacity values for various traffic conditions and scenarios. One example of this approach is an FHWA sponsored research report, Development of Highway Capacity Manual Methodologies To Assess Advanced Traffic Management Strategies That Influence Traffic Demand, which presents a methodology using different demand/capacity scenarios to develop travel time estimates that can be used to support the analysis of active transportation and demand management (ATDM) strategies’ impacts. (Dowling, R. Development of Highway Capacity Manual Methodologies To Assess Advanced Traffic Management Strategies That Influence Traffic Demand. Federal Highway Administration, U.S. Department of Transportation, Washington, D.C., 2011.) To predict the variability of demand in a project area, historical demand data is collected. The demand scenarios utilized in the report include 5 percent, 20 percent, 50 percent, 80 percent, and 95 percent demand scenarios. Additionally, capacity scenarios are generated. The capacity scenarios are constructed from historic data on incident and weather data for the facility (or facilities). After evaluating various scenarios with different combinations of capacity and demand values, the analyst determines a set of demand and capacity adjustment factors as shown in Tables 74 and 75. (Dowling, R. Development of Highway Capacity Manual Methodologies To Assess Advanced Traffic Management Strategies That Influence Traffic Demand. Federal Highway Administration, U.S. Department of Transportation, Washington, D.C., 2011.) These factors can be applied to the original model volumes and demands to adjust for particular mobility conditions.
Table 74. Example Scenario Demand Adjustment Factors
Demand Factors |
Demand Scenarios |
Capacity Scenarios |
5th Percentile |
20th Percentile |
50th Percentile |
80th Percentile |
95th Percentile |
Fair Weather, No Incidents |
0.88 |
0.93 |
0.96 |
0.99 |
1.01 |
Fair Weather, Single Lane Closed |
0.88 |
0.93 |
0.96 |
0.99 |
1.01 |
Fair Weather, 2 plus Lanes Closed |
0.88 |
0.93 |
0.96 |
0.99 |
1.01 |
Poor Weather, No Incidents |
0.88 |
0.93 |
0.96 |
0.99 |
1.01 |
Poor Weather, Single Lane Closed |
0.88 |
0.93 |
0.96 |
0.99 |
1.01 |
Poor Weather, 2 plus Lanes Closed |
0.88 |
0.93 |
0.96 |
0.99 |
1.01 |
(Source: Dowling, 2011.)
Table 75. Example Scenario Capacity Adjustment Factors
Capacity Factors |
Demand Scenarios |
Capacity Scenarios |
5th Percentile |
20th Percentile |
50th Percentile |
80th Percentile |
95th Percentile |
Fair Weather, No Incidents Present |
1.00 |
1.00 |
1.00 |
1.00 |
1.00 |
Fair Weather, Single Lane Closed
(Capacity Adjustment Factor = 1.00 – Proportion Capacity Reduction. For incidents, capacity adjustment factor is applied only to section and time slices where and when incident is present. For weather, capacity adjustment factor is applied to all sections and time slices.) |
0.58 |
0.58 |
0.58 |
0.58 |
0.58 |
Fair Weather, 2 plus Lanes Closed
(Capacity Adjustment Factor = 1.00 – Proportion Capacity Reduction. For incidents, capacity adjustment factor is applied only to section and time slices where and when incident is present. For weather, capacity adjustment factor is applied to all sections and time slices.) |
0.25 |
0.25 |
0.25 |
0.25 |
0.25 |
Poor Weather, No Incidents Present |
0.93 |
0.93 |
0.93 |
0.93 |
0.93 |
Poor Weather, Single Lane Closed
(Capacity Adjustment Factor = 1.00 – Proportion Capacity Reduction. For incidents, capacity adjustment factor is applied only to section and time slices where and when incident is present. For weather, capacity adjustment factor is applied to all sections and time slices.) |
0.51 |
0.51 |
0.51 |
0.51 |
0.51 |
Poor Weather, 2 plus Lanes Closed
(Capacity Adjustment Factor = 1.00 – Proportion Capacity Reduction. For incidents, capacity adjustment factor is applied only to section and time slices where and when incident is present. For weather, capacity adjustment factor is applied to all sections and time slices.) |
0.18 |
0.18 |
0.18 |
0.18 |
0.18 |
(Source: Dowling, 2011.)
This methodology allows analysts to evaluate the impacts and benefits a variety of ATDM strategies that may be considered to mitigate the impacts of various work zone configurations and construction alternatives. This also ensures that the analyst’s model of the work zone is calibrated to the specific demand and capacity values expected in the field for particular scenarios. Adjustments of the demand and capacity values enable analysts to attain improved confidence for their calculated travel times and other performance measures.
Travel Demand Diversion
A sensitivity analysis also can be used for estimating and/or evaluating trip diversion due to the work zone. One such tool, NETZONE, has the ability to estimate demand diversion based on different work zone project types and strategies. In NETZONE, the demand diversion model, which estimates the reduction in total demand and the diversion to other routes due to the construction work, is developed based on data from multiple work zone projects under different travel conditions (i.e., weekday, weekend, peak, off-peak, etc.) in California. (Zhang, M., W. Shen, Y. Nie, and J. Ma. Integrated Construction Zone Traffic Management. California PATH Research Report UCB-ITS-PRR-2008-9, 2008. Accessed January 11, 2012.)
With NETZONE an analyst may have to apply the demand diversion model. Several scenarios can be run using the demand diversion model. NETZONE was run on a case study for the SR 41 corridor network in Fresno, California. The three scenarios analyzed included:
- Scenario I – Before construction;
- Scenario II – During construction (do nothing scenario); and
- Scenario III – During construction with pre-trip information and media campaign.
The results of the demand diversion model provide general statistics about the facility or project. These statistics include total cost, delay, average cost, and maximum delay, as shown in Table 76. (Zhang, M., W. Shen, Y. Nie, and J. Ma. Integrated Construction Zone Traffic Management. California PATH Research Report UCB-ITS-PRR-2008-9, 2008. Accessed January 11, 2012.) This analysis can be used to gain further insight on how variations in demand affect mobility measures and costs. Additionally, this analysis also can be used to compare and select among various alternatives or scenarios.
Table 76. NETZONE Example General Statistics
Scenario |
Total Cost
(Hour) |
Total Delay
(Hour) |
Average Cost
(Hour) |
Maximum Delay
(Hour) |
I |
11,560 |
3,796 |
0.0049 |
0.1783 |
II |
13,303 |
5,232 |
0.0054 |
0.9083 |
III |
12,378 |
4,659 |
0.0052 |
0.9156 |
(Source: Zhang, Shen, Nie, and Ma, 2012.)
Sensitivity Analysis in Model Validation and Calibration
This section provides an overview on the use of sensitivity analysis to verify model outputs, as well as the accuracy or credibility of the model results. The sensitivity analysis also can be used to identify potential errors in model analysis outputs and/or MOEs.
The FHWA Report Traffic Analysis Toolbox Volume III noted that a sensitivity analysis was recommended to assess the reliability of microsimulation results. (Dowling, R., A. Skabardonis, and V. Alexiadis. Traffic Analysis Toolbox Volume III: Guidelines for Applying Traffic Microsimulation Software. Publication FHWA-HRT-04-040, Federal Highway Administration, U.S. Department of Transportation, Washington, D.C., June 2004.) The sensitivity analysis for this effort could include varying certain model parameters or changing the demand levels to determine what the impact may be on the simulation results. Some examples of its use include determining driving behavior parameter values that will best fit the project and the area. Impacts can be compared to field observations to verify what the parameter values should be. As a result of the sensitivity analysis, parameters can be customized to the work zone site characteristics, instead of using defaults. This helps to ensure the accuracy of the model outputs and analysis results.
VISSIM Example
A study by Chatterjee, et al. (2009) from the University of Missouri-Columbia involved a sensitivity analysis testing combinations of VISSIM model parameter values in order to determine the appropriate values for particular work zone configurations. (Chatterjee, I., P. Edara, S. Menneni, and C. Sun. Replication of Work Zone Capacity Values in a Simulation Model. In Transportation Research Record: Journal of the Transportation Research Board, No. 2130, Transportation Research Board, National Research Council, Washington, D.C., 2009, pages 138-148.) The study evaluated combinations of three different parameter values for several work zone configurations such as a two- to one-lane work zone and a three- to two-lane work zone. The three parameters included:
- CC1 – Desired time headway. This parameter was evaluated between the values of 0.9 to 1.8 seconds in increments of 0.1 second.
- CC2 – This parameter corresponds to the threshold that restricts longitudinal oscillation beyond a certain safety distance that varied. The parameter was evaluated between the values of 15 to 60 feet at increments of 5 feet.
- Safety Distance Reduction (SRF) Factor – This parameter refers to the reduction in safety distance to the trailing and leading vehicle in the desired lane and the safety distance to the leading vehicle in the current lane. This parameter was evaluated from the default value of 0.6 to 0.15 at increments of 0.05.
Scenarios of different combinations of the three parameters for each of the work zone configurations were generated and modeled using VISSIM (version 4.3). Note that the parameters and their values may vary in more recent versions; however, the following methodology should still be applicable for determining work zone capacity. Outputs such as occupancy and delay performance were collected to compare the different scenarios. The scenarios or combinations where model occupancy volumes exceeded the occupancy thresholds (determined through field occupancy data) were discarded from the analysis results. The end product of this sensitivity analysis included look-up tables that show the appropriate parameter values for different work zone configurations. In order to use the look-up tables, an analyst must first determine where their project or work zone alternative fits on a lane distribution plot based on the percentage of trucks in the field and work zone configuration. In this plot, an analyst would find the index number that best corresponds with the estimated capacity and percent of traffic on the right lane of their project. An example lane distribution plot is shown in Figure 33. (Chatterjee, I., P. Edara, S. Menneni, and C. Sun. Replication of Work Zone Capacity Values in a Simulation Model. In Transportation Research Record: Journal of the Transportation Research Board, No. 2130, Transportation Research Board, National Research Council, Washington, D.C., 2009, pages 138-148.) If an agency has a 2 to 1 configuration with a 5 percent truck percentage on the field, their analyst(s) can use this figure to determine the appropriate model parameter values to use when conducting the simulation. If the project area has a proportion of 11 percent for right lane traffic and a capacity of 1,550 vphpl, the lane distribution plot shown in Figure 33 shows that the index number associated with their project is number 19.
Figure 33. Lane Distribution at 1,000 Feet Upstream of Taper for Five Percent Trucks in a Two to One Configuration
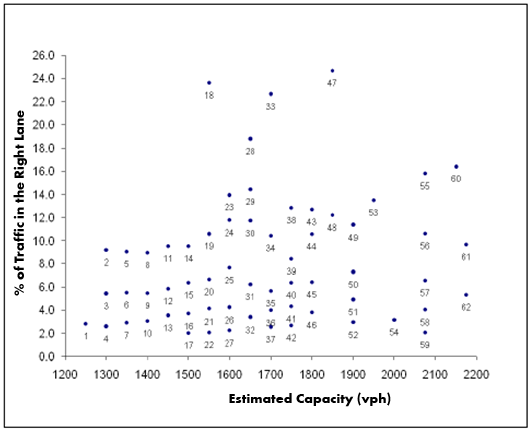
(Source: Chatterjee, Edara, Menneni, and Sun, 2009.)
In the next step, the analyst would then refer to the look-up tables shown on Figure 34 to find the corresponding CC1, CC2, and SRF factor parameter associated with index number 19. (Chatterjee, I., P. Edara, S. Menneni, and C. Sun. Replication of Work Zone Capacity Values in a Simulation Model. In Transportation Research Record: Journal of the Transportation Research Board, No. 2130, Transportation Research Board, National Research Council, Washington, D.C., 2009, pages 138-148.) According to the table, the parameter values associated with this example project are: 1) CC1 of 1.4; 2) CC2 of 40; and 3) SRF factor of 0.55.
Figure 34. Parameter Combinations for Two- to One-Lane Configuration
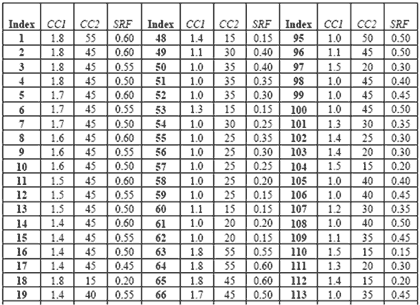
(Source: Chatterjee, Edara, Menneni, and Sun, 2009.)
Alternatives Analysis – Decision-Making and Performance Monitoring
This section describes how sensitivity analysis can be used in the decision-making process for choosing among different work zone alternatives or for determining which strategies and/or mitigation measures can be used to maintain a certain level of mobility across the work zone area. The following lists examples of these uses:
- Sensitivity analysis could be used to determine the impacts of varying the number of lanes closed, extent of closures, and the duration of construction;
- Sensitivity analysis also can be used to determine the optimal methods for dissemination of travel information, as well as for evaluating the impacts of this mitigation strategy on mobility; and
- Sensitivity analysis can be conducted to determine the impacts if traveler information was disseminated within 5 minutes of an incident occurring at the work zone versus 10 minutes or 15 minutes.
For choosing amongst alternatives, sensitivity analysis can be used to:
- Determine a threshold or range that the project must meet in order to achieve the construction schedule or financial objectives; and
- Determine the sensitivity of total project costs to various measures and project characteristics such as traffic flow rates and work zone length.
An example of how sensitivity analysis can be used for determining optimal work zone alternatives is a study by Tang and Chien (2009) where the researchers developed a model for optimizing work zone schedules jointly considering the time-varying traffic diversion, variable maintenance cost, and production rate of different maintenance crews. (Tang, Y., and S. Chien. Optimization of Work Zone Schedule Considering Time-Varying Traffic Diversion. The 89th TRB Annual Meeting, National Research Council, Washington, D.C., Paper No. 10-0184, January 2010.) The authors’ model identifies the total cost (agency and user cost) associated with different combinations of these factors. An agency may use such a model to plan maintenance activities cost-effectively and choose among different work zone alternatives. This model optimizes a work zone schedule based on two modules:
- Diversion module that determines the time-varying traffic diversion; and
- Genetic Algorithm (GA) module that uses the redistributed traffic flows from the Diversion module in order to generate improved work zone schedules and update the initial/previous work zones schedules for the next iteration.
The work zone schedule goes through several iterations of Modules 1 and 2 until an optimal schedule is achieved when a threshold, in this case the minimized total cost, is reached.
This approach was applied on a resurfacing project in New Jersey. The analysis served to analyze different combinations of scenarios, including the following:
- Scenario A without traffic diversion; and
- Scenario B with traffic diversion.
Additionally, the analysis applied four potential maintenance crews with varying maintenance costs and production times to the two scenarios, which brought the total number of scenarios to eight as shown in Figure 35. (Tang, Y., and S. Chien. Optimization of Work Zone Schedule Considering Time-Varying Traffic Diversion. The 89th TRB Annual Meeting, National Research Council, Washington, D.C., Paper No. 10-0184, January 2010.) The table also summarizes the combined effects of traffic diversions and different construction methods on the project total cost.
Figure 35. Summary of Cost Comparison for Scenarios and Maintenance Crews
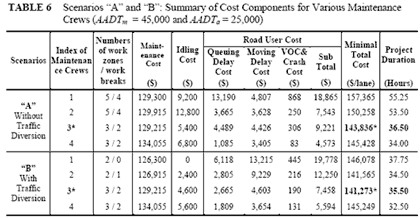
(Source: Tang and Chien, 2010.)
After the scenarios were developed, a sensitivity analysis was conducted in order to evaluate the benefit of traffic diversion by varying the mainline AADT (AADTm) incrementally from 30,000 vpd to 60,000 vpd for both Scenarios A and B. The minimized total costs associated with the sensitivity analysis are shown in Figures 27 and 28. The sensitivity analysis showed that in comparing maintenance crews 1 and 2 (Figure 27), diverting traffic proved to be the most economical strategy if less productive maintenance crews were employed for work zones on heavily traveled highways. However, it also showed that a more productive maintenance crew would be just as cost-effective if traffic was not able to divert to alternate routes.
Figure 36. Sensitivity Analysis Results for Crews 1 and 2
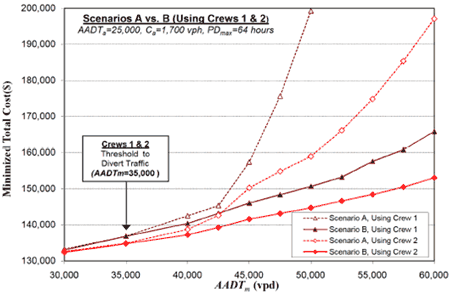
(Source: Tang and Chien, 2010.)
Figure 37. Sensitivity Analysis Results for Crews 3 and 4
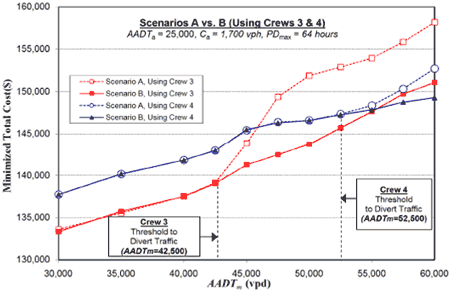
(Source: Tang and Chien, 2010.)
PDF files can be viewed with the Acrobat® Reader®.